spctoolkit
by Donald J. Wheeler
Charts for Rare Events
Counts of rare events are inherently insensitive and weak.
Your imagination is the only limitation on the use of Shewhart's charts.
They are such a basic tool for analyzing and understanding data that they
can be used in all kinds of situations-the key is to see how best to apply
them. This column will illustrate how to use Shewhart's charts to track
rare events.
Department 16 has occasional spills. The most recent spill was on July 13.
Spills are not desirable, and everything possible is done to prevent them;
yet they have historically averaged about one spill every seven months.
Of course, with this average, whenever they have a spill, they are 690 percent
above average for that month. When dealing with very small numbers, such
as the counts of rare events, a one-unit change can result in a huge percentage
difference.
Counts of rare events would commonly be placed on a c-chart. (While the
c-chart is a chart for individual values, and while most count data may
be charted using an XmR chart, the XmR chart requires an average count that
exceeds 1.0. The c-chart does not suffer this restriction.) The average
count is found by dividing the total number of spills in a given time period
by the length of that time period. During the past 55 months, a total of
eight spills occurred, which gives an average count of:
c bar = 8 spills/55 months = 0.145 spills per month
This average will be the central line for the c-chart, and the upper control
limit will be computed according to the formula:
UCLc = c bar + 3 (square root of c bar ) = 0.145 + 3 (square root of 0.145
) = 1.289
The c-chart is shown in Figure 1. In spite of the fact that a single spill
is 690 percent above the average, the c-chart does not show any out-of-control
points. This is not a problem with the charts but rather a problem with
the data. Counts of rare events are inherently insensitive and weak. No
matter how these counts are analyzed, there is nothing to discover here.
Yet there are other ways to characterize the spills. Instead of counting
the number of spills each year, they could measure the number of days between
the spills. The first spill was on Feb. 23, Year One. The second spill was
on Jan. 11, Year Two. The elapsed time between these two spills was 322
days. One spill in 322 days is equivalent to a spill rate of 1.13 spills
per year.
The third spill was on Sept. 15, Year Two. This is 247 days after the second
spill. One spill in 247 days is equivalent to a spill rate of 1.48 spills
per year. Continuing in this manner, the remaining five spills are converted
into instantaneous spill rates of 1.24, 1.61, 1.64, 2.12 and 3.17 spills
per year. These seven spill rates are used to compute six moving ranges
and are placed on an XmR chart in Figure 2.
The average spill rate is 1.77 spills per year, and the average moving range
is 0.42. These two values result in an upper natural process limit of:
UNPL = 1.77 + 2.66 x 0.42 = 2.89
the lower natural process limit will be:
LNPL = 1.77 ­p; 2.66 x 0.42 = 0.65
and the upper control limit for the moving ranges will be:
UCL = 3.268 x 0.42 = 1.37
This XmR chart for the spill rates is shown in Figure 2.
The last spill results in a point that is above the upper natural process
limit, which suggests that there has been an increase in the spill rate.
This signal should be investigated, yet it is missed by the c-chart.
In general, counts are weaker than measurements. Counts of rare events are
no exception. When possible, it will always be more satisfactory to measure
the activity than to merely count events. And, as shown in this example,
the times between undesirable rare events are best charted as rates.
About the author
Donald J. Wheeler is an internationally known consulting statistician
and the author of Understanding Variation: The Key to Managing Chaos,
Advanced Topics in Statistical Process Control and Understanding
Statistical Process Control, Second Edition.
© 1996 SPC Press Inc. Telephone (423) 584-5005. E-mail: dwheeler@qualitydigest.com.
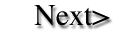