by Douglas C. Fair
Most manufacturers have heard of Six Sigma and its quality improvement methodologies designed to dramatically reduce defects and costs. Although Six Sigma can be applied without technology, most companies choose to leverage the methodology's effectiveness through computers and software.
Consider the Six Sigma cycle of define, measure, analyze, improve and control (DMAIC). Throughout the different stages, a variety of tools and statistical methods are used. During the analyze phase, one might use regression analysis and hypothesis testing. Design of experiments (DOE) techniques, such as full and fractional factorial experiments, might also be applied. Statistical process control (SPC) techniques might be employed for the control portion of the DMAIC cycle.
As Six Sigma and lean initiatives push the responsibility for reducing process variability and increasing product reliability into the hands of shop floor employees, the need for intuitive and effective shop floor SPC software has grown substantially.
Particularly for SPC, the right statistical software can greatly ease the burden of data collection, analysis and real-time monitoring. Newer software packages have user interfaces that make shop floor deployment much simpler and ease the amount of training required for operators to effectively use the data to control their processes in real time. For instance, you might typically see real-time SPC software used on the shop floor by machine operators to control processes and signal changes that might cause problems in the near future or to immediately trigger an alarm for an out-of-control process.
Although both DOE and SPC are statistical tools, each has unique functions, users and focus. For example, DOE is used to identify primary variables and their effects on process performance and is generally utilized by quality professionals in off-line situations--that is, when the manufacturing process isn't producing marketable product.
On the other hand, SPC is an online statistical tool typically used on the shop floor by machine operators to control processes and signal changes that might cause problems in the near future. Therefore, SPC isn't only an online statistical tool but also a real-time one because alarms can be triggered instantaneously the moment data are entered.
Because of their vast differences--both philosophically and in everyday use--online and off-line statistical software solutions aren't usually packaged together. Thus, if you want to conduct real-time online SPC as well as off-line analysis, you'll need at least two software packages.
The following case study shows how a carton manufacturing plant used SPC software to solve problems and improve its processes.
Just a few years ago, a large folding-carton plant (let's call it "Plant A") in the southeastern United States faced an enormous challenge. When compared with other plants in its company's division, Plant A had the worst quality and performance metrics, and its customers were unhappy. Very unhappy. In fact, Plant A's two largest customers had sent letters confirming that if action wasn't taken to improve carton quality, they would remove their business from the plant.
In just six months everything changed as management began the process that would transform Plant A into the high performer it is today. Dramatic improvements in product quality and productivity fueled Plant A's rise to leadership in all quality and performance categories in the entire division. The gains were impressive and fast. When asked what impression it had made on its customers, Jeff, the plant manager, offered this curious response: "They don't even know who we are." It was a baffling answer for a plant that ships more than 50 million beverage cartons each month to industry giants such as The Coca-Cola Co., Anheuser-Busch Companies Inc. and others.
Conventional thinking would assume that such significant improvements in quality would exhilarate a vendor's customers. At the very least, it should entice their curiosity. Surely their customers must be happy to have been on the receiving end of such significant quality improvements. "Well, yes, they were happy," Jeff acknowledged. "But in the folding-carton business, the only time customers actually know who their supplier is, is when the supplier makes bad cartons. They don't know us anymore because we've achieved our goal of becoming completely anonymous."
Beginning the process When Jeff and his team were installed at Plant A, what they found was fairly common. Plant A was a mix of old and new technologies, high scrap levels and a towering customer complaint record. Because of these complaints, improving carton quality quickly became a top priority. Customer complaint data were readily available and instructive but did little to focus process improvement efforts. "We knew we had problems," Jeff explained, "but we just didn't have internal visibility to catch or predict quality issues before we heard about them from our customer."
The team could have started data collection and problem solving in any of the plant's primary processes: sheeting, printing, cutting or finishing. But the team wanted to focus its efforts and maximize improvements in a short time by achieving two objectives simultaneously:
1. Prevent defective cartons from being shipped to customers.
2. Gather data that would fuel product quality improvement.
The team didn't want simply to pick the most likely problem area to begin its improvement activities. Instead, it wanted data to drive improvement activities. To this end, the team adopted a statistical sampling strategy devised to collect data at the back door of the manufacturing operation. That is, product quality data would be gathered after all manufacturing operations were completed but just before the cartons were palletized and shipped from the back door of the plant. Prior to palletizing, samples were inspected for defects, and if any were found, they were logged into the plant's real-time SPC software. If certain statistical defect levels were reached, the entire pallet was placed on hold and the shipment stopped.
Back-door data collection effectively ended the flow of defective cartons, allowing the Plant A team to achieve its first goal while also providing information regarding the most prevalent carton defects. This information led to process and product quality improvement, which allowed Plant A to achieve its second goal. How the plant achieved this is explained below. Although the specific data have been changed to protect Plant A's competitive position, the situations and examples showing how the company achieved improvement have not.
The back-door data-collection plan allowed management to view the frequency of the defect codes on a single chart (as seen in figure 1 at the upper left). Surprisingly, the resulting Pareto chart indicated that the most common defects weren't cosmetic or print-related. Instead, most carton defects proved to be folded, bent or damaged carton flaps.
When encountered at Plant A's customers' sites, functional defects can jam carton-filling equipment and force expensive machine downtime. Not only were machine shutdowns exasperating for its customers, Plant A's expenses were affected as well, due to customer credits and returns. The company's management knew that to reach the plant's goal of becoming "nameless" to its customers, it needed to stop defects from reaching customers and prevent defects from occurring in the first place.
While performing back-door data collection, inspectors noted not just the defect code but the production line from which it originated. To identify specific actions that could be taken at each line to reduce defects, they created a multilevel Pareto chart (as seen in figure 2 on page 31). This type of Pareto chart displays the identical defects found in figure 1 but sorts them by production line. Each line's defects are summarized and displayed in a yellow bar, while the blue bars illustrate frequency and type of defects. The overall result is a Pareto chart within a Pareto chart.
In figure 2, each line's specific defect codes helped management allocate improvement resources and identify unique changes to each line that would reduce the plant's overall functional defects.
Reducing complaints Once the changes were successful in reducing the primary defects on each line, management chose to evaluate the next largest defect: cartons that wouldn't open. These defective cartons at a customer site result in runability problems with filling machines, which means more downtime as setups and settings are changed. To better understand how to reduce these defects, Plant A targeted carton-opening force as a critical parameter that should be controlled. Opening force is the amount of pounds per square inch (PSI) required for opening a flat container so that it can be filled with beverage cans. Opening force can only be checked after a carton has gone through all stages of carton manufacturing, including the folding and gluing process. Therefore, the Plant A team began collecting opening-force data on finished cartons on each of its folder-gluer machines.
To understand how folder-gluers were performing, a "deviation from target" control chart was used to evaluate opening force. Although each product may have different opening-force specifications, the chart is normalized to accommodate unique customer tar--get values. The chart allows statistical control of the process, regardless of the cartons being manufactured.
After incorporating the deviation-from-target control chart, operators and quality managers found that opening force varied dramatically over time, from one job to the next and from shift to shift (as seen in figure 3). This resulted in numerous statistical alarms on both the X-bar and range charts. Additionally, the average opening force was almost always greater than the customer target values, represented by a red horizontal line on the X-bar chart. After working with engineers and operators, the plant instituted changes to reduce the average opening force. It instituted procedures to minimize differences between shifts, jobs and other process variables. The result was significantly less opening-force variation and average values much closer to customer targets (as seen in figure 4). In a very short time, customer complaints about opening force were virtually eliminated.
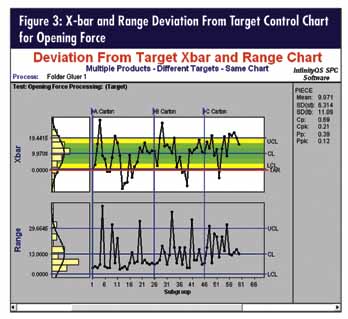
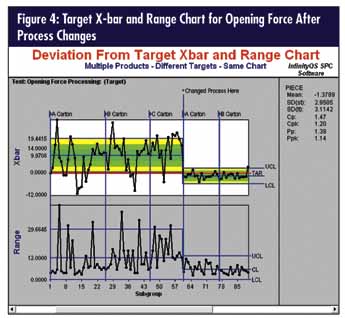
Additionally, Plant A's customers were able to use a single, simplified setup of filling machines, reducing complexity and setup time.
"We needed to give our customers a consistent product so they could run their packaging machines without stopping," says Plant A's quality assurance coordinator. "If we send them a more reliable product that runs well for them, then it not only costs them less money, but it costs us less as well because we don't have to go on-site to fix the problems."
After functional defects and opening force, the next several bars on figure 1's Pareto chart indicate poor ink adhesion, off-color, ink smears and streaks, oil spots, fisheyes and missing color. All these defects are related to the carton printing process. Engineers and operators noted that many of the print-related defects resulted from scumming, a problem that can occur in the fountain solution of printing presses. It's one of the primary sources of print-related defects.
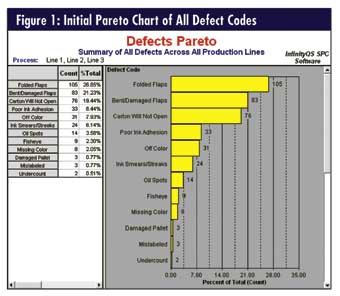
Plant A's operators needed to attack the pH of the fountain solution and conductivity at the printer. If these two critical parameters aren't precisely controlled, they can lead to scumming, which in turn creates print defects. Operators periodically take a fountain solution sample, check pH and conductivity, and record results on control charts. To evaluate pH, they plot data values and analyze them on an individual X and moving-range control chart. To apply a control chart to conductivity was more challenging. Because conductivity is naturally cyclical, a standard control chart with linear control limits couldn't be applied. Plant A's operators needed a tool that would model the natural tendency for conductivity values to slowly increase over time. They chose the exponentially weighted moving average (EWMA) control chart with wandering mean. This specialized chart is particularly useful for tracking chemical processes, tool wear or any situation where data values are expected to gradually increase or decrease over time, rather than adhere to a linear data model.
The EWMA chart incorporates a "next step predictor" based on previous subgroups of data. If previous plot points increase incrementally, then the EWMA chart predicts the next value based on historical data. Using this prediction, control limits change and create a "stepped" appearance, such as the one exhibited by the control chart in figure 5.
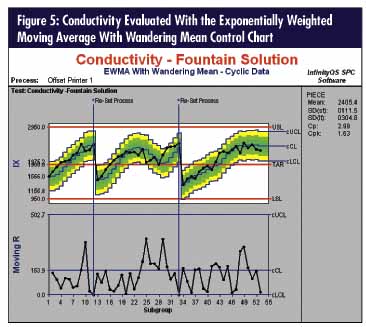
The EWMA control chart with wandering mean helped operators assess the control of individual subgroup values while simultaneously determining when to reset the fountain solution before it exceeded specification limits. By more closely controlling both pH and conductivity, print operators were able to control and reduce scumming, which resulted in a significant drop in print-related defects.
In addition to using its SPC software for the data collection and analysis described above, Plant A also used it to log customer feedback information into the system. For instance, when a carton die is run again, historical defects and customer comments from the previous run can be viewed by the operator and compared to the newest job. Past and current results can be compared with each other in real time. Assignable causes and corrective actions are also logged into the system to further enhance operator knowledge and communicate to others how best to run containers to minimize defects and ensure carton consistency.
"Because of the information logged into the software, we don't need to call our customers to understand what happened the last time we ran the job," notes Bob, the plant's quality coordinator. "Operators are instantly aware of any problems that occurred in the previous run. This benefits our customers as well as our own upstream processes. The finishing operators can view defects downstream of their process and vice versa, resulting in far better communications within the plant."
Much coordinated hard work and effort was applied to systematize SPC and make measurable and continuous improvement a part of Plant A's Six Sigma efforts. Although the spectacular results and improvements early in the implementation have leveled off, operators, engineers and managers continue to use SPC. Even with a new plant manager, Plant A has continued to advance the quality improvement efforts implemented years ago and has improved from last place in quality to first place in its division. Other results include:
• Six Sigma levels of quality achieved on primary quality characteristics
• Dramatic reduction in defect levels
• Elimination of customer quality concerns
• Lowest parts per million (PPM) defective rate in the entire division
• Lowest customer complaints per million cartons produced
Through the diligent, ongoing and consistent use of SPC and Six Sigma to improve carton quality, Plant A has seen dramatic reductions in defect rates, customer complaints and internal costs. At the same time, the plant has achieved coveted key-supplier certification from customers that were prepared to walk away only months before.
Although the largest problems have long since been solved, operators still use SPC to monitor processes and ensure consistency and quality. In other words, SPC is still used for the "control" phase of the Six Sigma DMAIC model.
"It's no longer a question of, 'Did we fix something?'" says Jeff. "Instead, it's a question of, 'Did it stay fixed?' As we can continue to control our processes with SPC, we will maintain our consistency and continue to be an unknown, anonymous vendor."
As vice president of statistical applications at InfinityQS International Inc., Douglas C. Fair helps clients understand statistical methods and implement InfinityQS software in diverse environments and challenging situations.
Fair has co-authored two books on statistical methods: Innovative Control Charting (ASQ Quality Press, 1997) and Principles and Methods of Quality Management in Health Care (Jones and Bartlett Publishing, 2000). He is a bimonthly columnist for Quality Digest's QualityInsider (www.qualitydigest.com/qualityinsider).
|