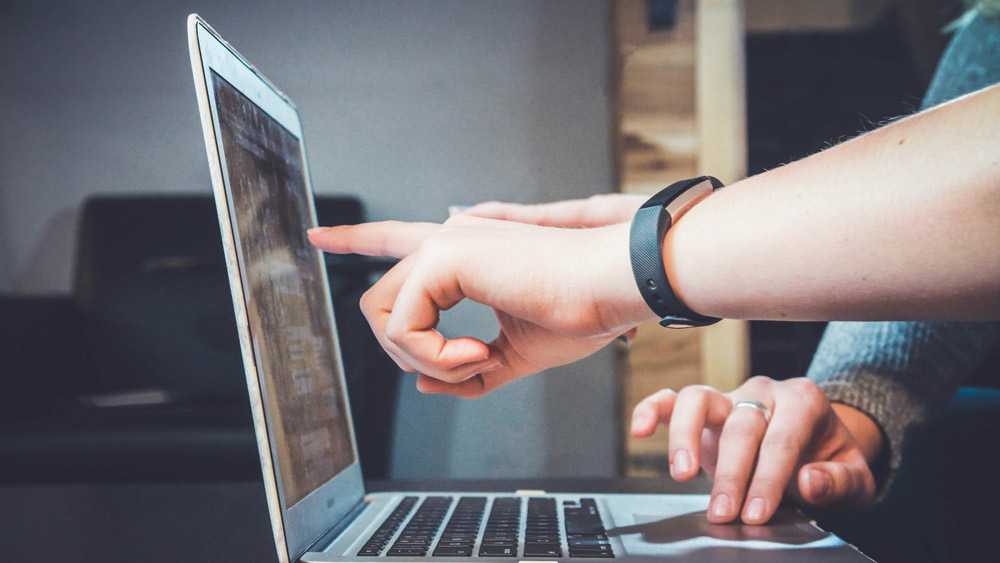
If data are currency, we must invest in tools that grow the investment and put data to work in the most effective way. Photo by John Schnobrich on Unsplash
W ith the rapid digital transformation of manufacturing, including technologies like cloud solutions, digital twins, next-generation devices, automation, and AI, the role of metrology is poised for a transformative evolution. What can we expect from new quality systems, and how is the metrology office’s purpose changing to tackle industrial opportunities and challenges?
ADVERTISEMENT |
Manufacturers are under pressure to bring better products to market more quickly and more sustainably than ever. Improving the quality of new products faster is a top priority.
But quality challenges remain prevalent. Unanticipated quality issues and poor product quality are common issues. This shows that the traditional quality control methods are no longer adequate, and we must accelerate away from a reactive approach and toward a proactive position on quality. Other factors, like time to market and pace of innovation, are also important.
…
Add new comment