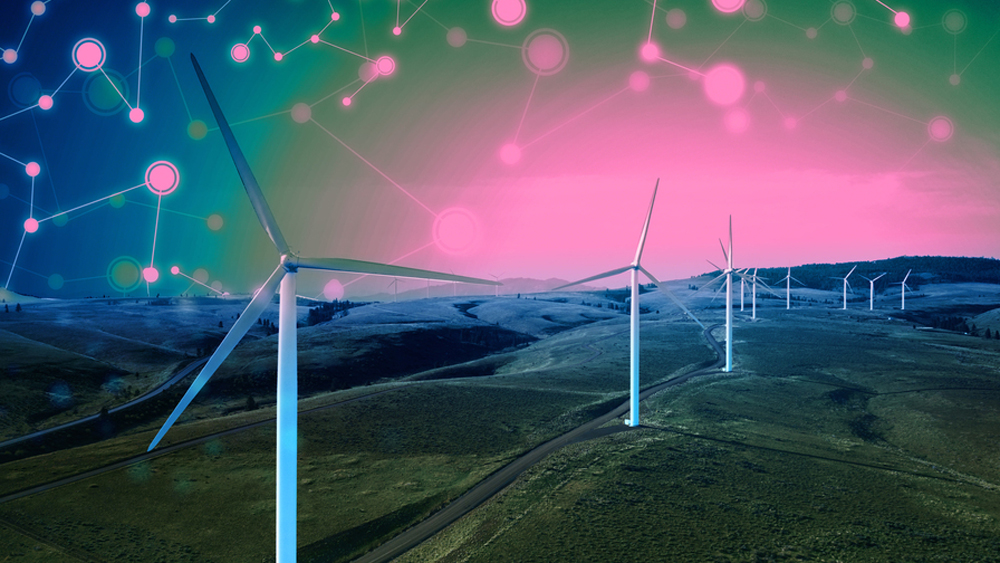
The new method could someday help alert technicians to potential problems in equipment like wind turbines or satellites. Credit: MIT News, iStock
Identifying one faulty turbine in a wind farm, which can involve looking at hundreds of signals and millions of data points, is akin to finding a needle in a haystack.
ADVERTISEMENT |
Engineers often streamline this complex problem using deep-learning models that can detect anomalies in measurements taken repeatedly over time by each turbine, known as time-series data.
But with hundreds of wind turbines recording dozens of signals each hour, training a deep-learning model to analyze time-series data is costly and cumbersome. This is compounded by the fact that the model may need to be retrained after deployment, and wind farm operators might lack the necessary machine-learning expertise.
In a new study, MIT researchers found that large language models (LLMs) have potential to be more efficient anomaly detectors for time-series data. Importantly, these pretrained models can be deployed right out of the box.
…
Add new comment