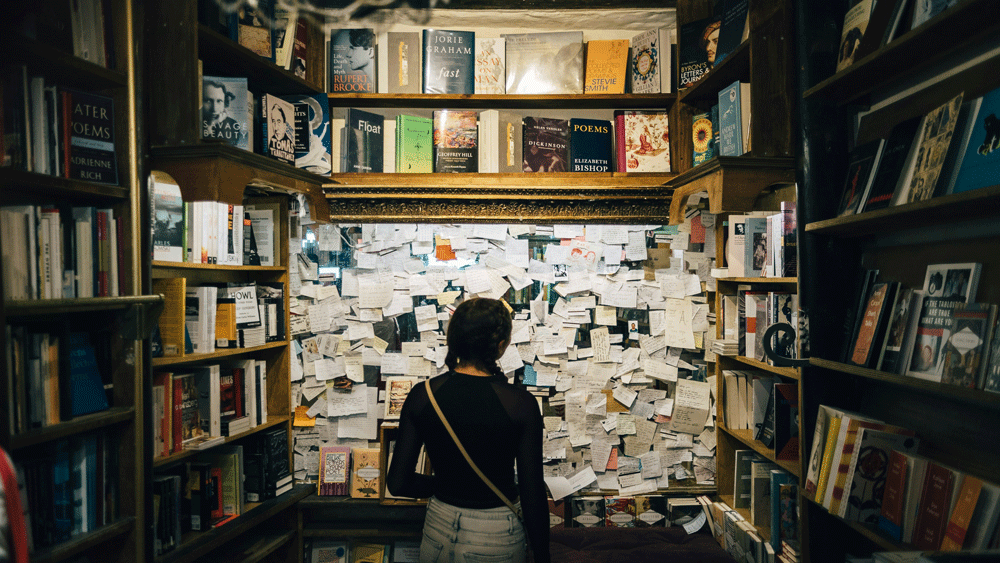
Photo by Brandon Lopez on Unsplash
Today I’m looking at the free energy principle (FEP) by the British neuroscientist Karl Friston. The FEP basically states that to resist the natural tendency to disorder, adaptive agents must minimize surprise. This has implications for the gemba, as you’ll see.
ADVERTISEMENT |
A good example to explain this is to say, “Successful fish typically find themselves surrounded by water, and very atypically find themselves out of water, since being out of water for an extended time will lead to a breakdown of homoeostatic (autopoietic) relations.”1
Here, the free energy refers to an information-theoretic construct:
“Because the distribution of ‘surprising’ events is in general unknown and unknowable, organisms must instead minimize a tractable proxy, which according to the FEP turns out to be ‘free energy.’ Free energy in this context is an information-theoretic construct that (i) provides an upper bound on the extent to which sensory data is atypical (‘surprising’); and (ii) can be evaluated by an organism, because it depends eventually only on sensory input and an internal model of the environmental causes of sensory input.”1
…
Add new comment