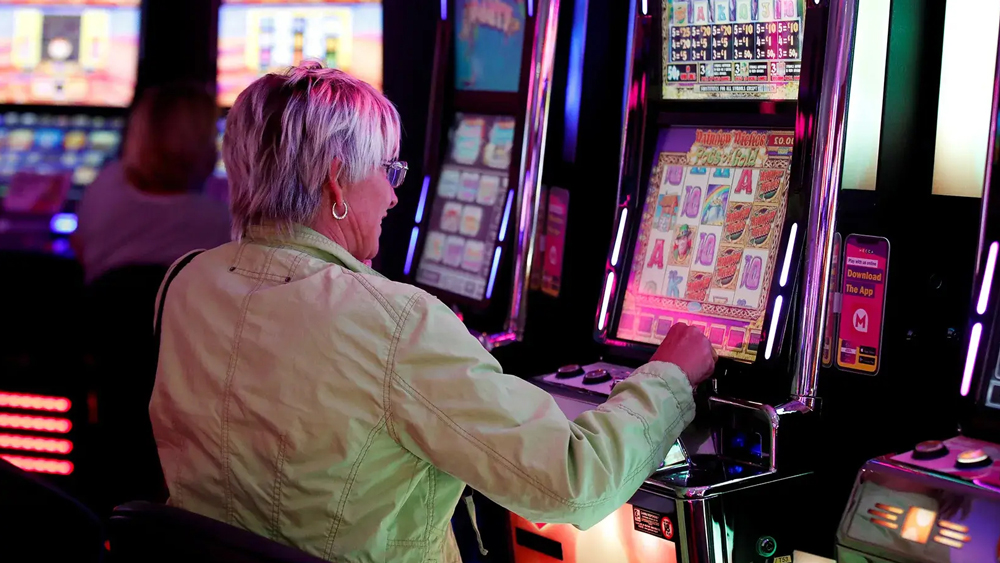
The concept of “bandit experiments” comes from the metaphor of a gambler facing a row of slot machines and choosing which arms to pull to win the most. Credit: Reuters/Peter Cziborra.
Researchers at Stanford Graduate School of Business have pushed past the limitations of A/B testing into another area of experimentation focused on “multi-armed bandits.” Mohsen Bayati, a professor of operations, information, and technology who has been exploring these problems for the past 15 years, explains the basics of this approach and how it can pay off.
ADVERTISEMENT |
What are multi-armed bandits?
You can think of multi-armed bandits as a class of decision-making scenarios individuals or algorithms face when choosing between multiple options with uncertain outcomes. The name comes from the metaphor of a gambler facing a row of slot machines and choosing which arms to pull in order to maximize their total payout. They don’t know which arm is best in advance, so they experiment. Once they figure out which arm is a good one, they want to stick with it.
…
Add new comment