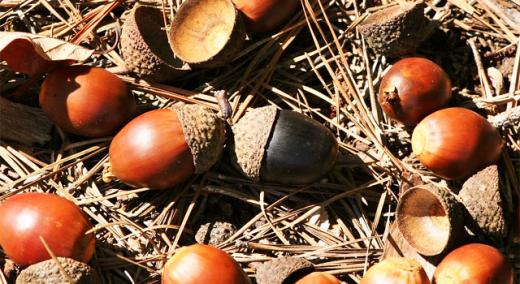
In manufacturing today, data analysis tools can give management the information it needs to make better decisions in areas such as maintenance and labor. Unfortunately, however, many data analytics systems require large sets of historical data to generate accurate and useful results.
ADVERTISEMENT |
According to Rebecca Grollman, a data scientist at Bsquare, anomaly detection is different. These algorithms can begin generating useful information without needing to be trained on historical data. Although simple, anomaly detection can be used for applications such as detecting machine stoppage, sensor malfunctions, tracking production output, and more. Engineering.com recently spoke with Grollman about this solution.
How essential is historical data in typical data science applications?
…
Add new comment