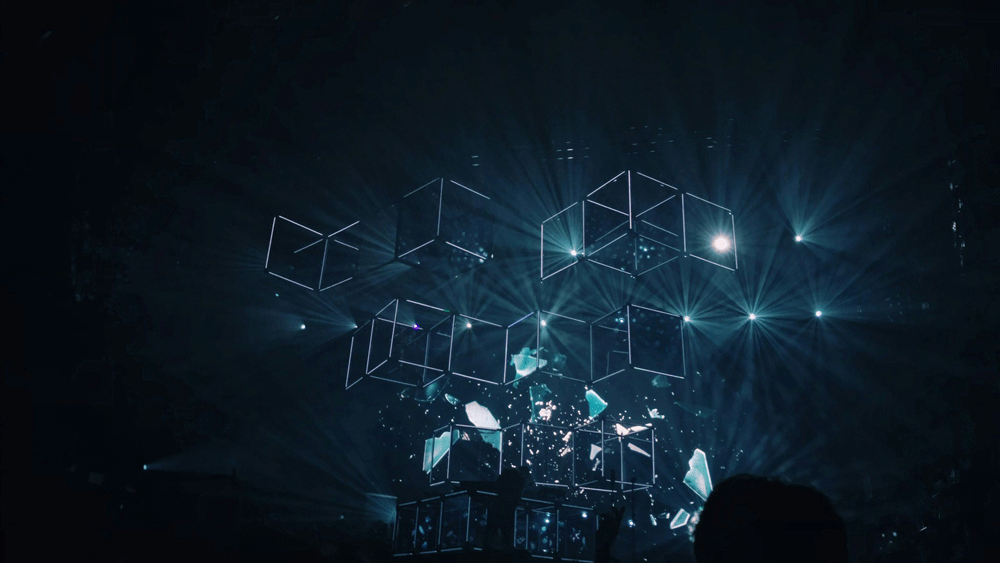
Data are the fuel of the Information Age, and all organizations acknowledge the value of well-managed corporate data assets. The problems start when we begin to ask just exactly how the data assets are to be well managed.
ADVERTISEMENT |
In response, many organizations have put data governance departments in place and have tasked them with ensuring good data management practices. That by itself still doesn’t solve the problem. A typical data governance unit might consist of five employees in a total workforce of 5,000, so you can immediately see the problem of how such a unit can scale its efforts to cover the entire enterprise.
Yet the mission is worthwhile. For instance, in quality management, the proper handling and curation of datasets produced by metrology is of great importance. But this is only one of many examples. Data privacy, data retention (purging outdated data), accurate specifications, and definitions of reported metrics are some of the other data management tasks requiring attention, and there are many more. Still, the question remains of how a data governance unit can scale to address all these needs.
…
Add new comment