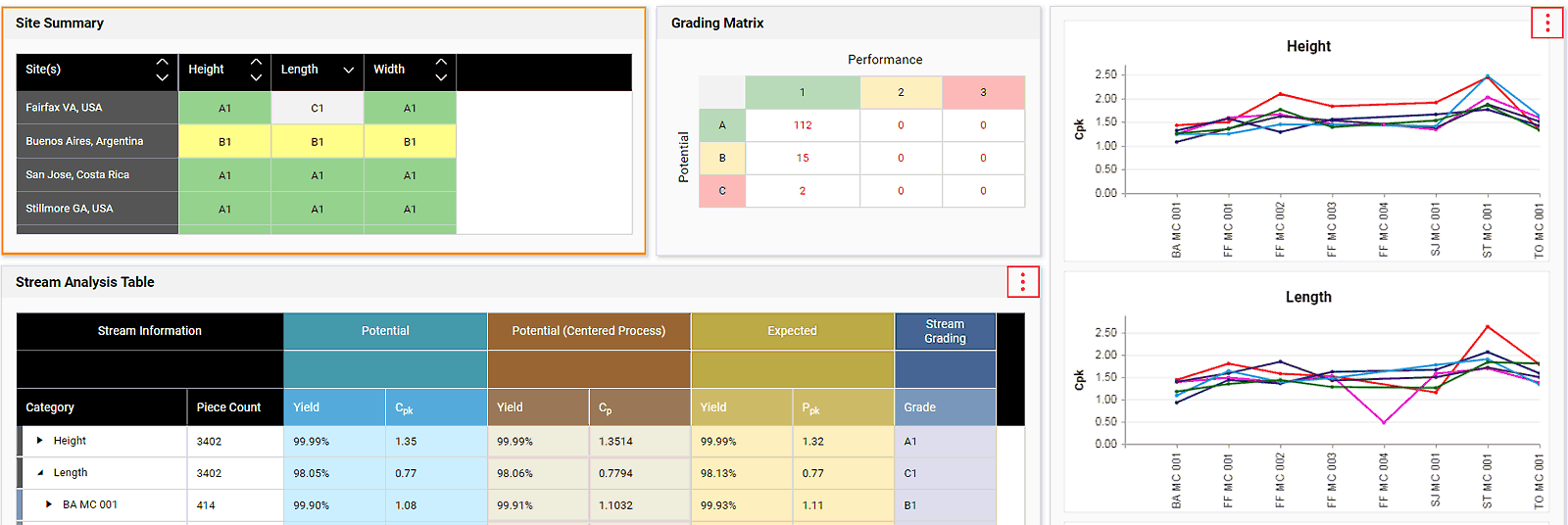
I recently sat down with Doug Fair, the chief operating officer of InfinityQS, for a discussion about the uses (and sometimes, the misuses) of industrial statistical analysis. Ours was a lengthy conversation, so we’re splitting the account into two parts. In this installment, we cover data gluttony and the power of sampling. In part 2, coming up in October, Fair and I will compare and contrast a pair of interesting case studies and consider what manufacturers are missing when they fail to consider the data obtained from in-spec processes.
Quality Digest: Modern manufacturers are suffering from an ailment known as “data gluttony.” Can you explain the meaning of that phrase?
Doug Fair: I’m an industrial statistician and an old-school quality guy with 31 years in this business. When I started out, we had analog gauges and data on pieces on paper. Now, with the wonderful advent of the technology we’re able to enjoy today, we have programmable logic controllers [PLCs] and OPC [OLE for process control] servers, databases and a wide variety of IT. All of this information technology can be deployed out on the shop floor, and we don’t need to use paper and pencil anymore. We now have these sophisticated and inexpensive technologies that can be used to gather virtually any data we want to gather. And that’s fantastic, because now we’re able to apply more science to quality control, process control, and performance improvements. The downside is that these data are so easy to gather that manufacturers I deal with say, “We want to gather it all.” But when I ask them, “Why do you want to gather it all?” they don’t have a good answer. Basically, they want to gather it all just because the data are available, and that’s not a good reason, nor is it an economical one.
So, I call a big time out on that. I think it’s great that companies have access to these data; however, without a plan for what to do with them, it’s going to turn into a massive problem. Data gluttony results in huge expenses to organizations, and a lack of a return on investment. The data has to go somewhere, so the end result is lots of expenditures on IT technologies like databases, servers, and security technologies, so when I hear companies saying that they have 43 different features, both process-specific and product-specific, and they’re getting data every few seconds or every few milliseconds, I just say, “Hey guys, if you’re collecting everything, then you really don’t know what you want.” That’s a problem, because if they don’t know what they want, if they’re not sure what visibility they need into their processes, then typically they settle for data gluttony—and data gluttony is very expensive and challenging to manage and support.
QD: Would you say that’s a legacy belief system? A lot of us grew up in an era where data were like a silver bullets, meaning that if data are great, more data must be greater! But maybe there’s a downside to that as well.
DF: Absolutely. Because if everything is a priority, then nothing is. As a statistician I know that if you’ve got 43 different features out there, generally your processes will follow the Pareto principle, where the vital few of those features drive the quality of that product, and the trivial many should be excised. If you’re gathering all those data, then companies need extensive IT systems. IT resources are very costly, so if you’re filling up hard drives quickly by gathering data every millisecond across an enterprise, it’s going to be very expensive to manage. That’s why data gluttony can prevent organizations from reducing their costs like they should be able to do with modern IT technologies. Plus, when you’re gathering all these data, the biggest downside is that there’s no focus on what truly is driving quality. Ultimately, the most successful organizations that I have worked with use data for very specific reasons: to improve manufacturing efficiencies and reduce overall cost of the products they make and processes used to make them.
I submit to you that, first, companies must stop data gluttony. Not everything should be measured, and not all data should be gathered. Organizations need to stop and think about what is truly needed to improve quality and operations. They need to identify the features that they truly need to gather data on, and they need to pare down the remainder. Then, once data have been gathered, they need to invest time in analyzing those data to uncover information that can help them make big improvements in cost, efficiency, and quality.
Second, data sampling is vitally important. I’ve seen companies that say, “Hey, we’ve got to monitor the temperatures in this die that we’re checking, and we want to gather data every few milliseconds.” No! Temperatures will not change significantly in that metal die over many seconds, even minutes, of time, so sampling is going to be where it’s at in terms of gathering data efficiently.
QD: If I’m understanding you properly, you’re talking about contextualization of data, and having the experience to understand your process well enough to know what the data are telling you. And it’s not actually data, necessarily, it’s information, because data are one thing, and information is another. You want the kind of information that will improve your process, rather than just saying, “We don’t know what we want, so we want everything.”
DF: I would say yes. I think manufacturers today have a data problem and an information deficit.
QD: Well put.
DF: They don’t want data. They really don’t. What they need is information. That’s where your statistical techniques and other data analysis aggregation and summary techniques come into play, by helping extract meaningful information from those data. So the data themseves are, quite frankly, meaningless except for their ability to drive information to us.
QD: What’s meant by the term “data interrogation?”
DF: I have never seen a data set that wasn’t chock-full of information... large, small, and everything in-between. Whenever people give me a whole bunch of individual data values in an Excel spreadsheet, as a statistician I’m blown away by the information that can be learned from those thousands or hundreds of data values. Shining a bright light on these individual data values through the use of statistical techniques allows us to summarize and extract the golden nuggets of information from those data—it’s what I like to call data interrogation.
The most successful organizations that our company works with make it a habit to step back from the daily grind, from the details of their data, on a regular basis. Perhaps once a week or once a month, managers, engineers, and quality professionals will meet and ask of one another, “OK, what can we learn from these important data that we’ve gathered?” They’ll look at these data in different ways, and interrogate the data using a variety of different statistical tools and techniques, and extract the most valuable information. That is, they regularly interrogate the data they have gathered.
I’ve seen organizations save millions of dollars through data interrogation. One company I’ve worked with, a 300-plus person plant, literally saved their plant by interrogating their data on a weekly basis. This improved quality outcomes enough that the plant was kept from being shut down. But here’s the thing: It’s all related to the issue of data gluttony. If you get a bunch of data, you must have a plan for what you will do with those data. If organizations just collect these data to check a box, or to say, “Hey, we collected that,” or worse, simply as a CYA exercise, then those are the wrong motivations for data collection.
I have dealt with companies that have literally saved tens of millions of dollars by stepping back and looking at their data on a frequent basis, and they’ve been blown away by the results. The plant I referenced earlier was on the precipice, and it was about to fall over the edge. Customers were telling them, “We are going to pull millions of dollars of contracts from you because your product quality is so bad.” The managers put together a sampling plan across the entire plant, and over a period of four months, they went from the lowest quality (from a PPM perspective throughout the enterprise), to the highest quality across their company’s enterprise. Data interrogation allowed them to go from worst quality to first quality. They saved their plant, and grew the business, and made their customers happy, too. In fact, I saw a framed letter that had been put up on a wall. It was from a client, who wrote, “We’re not sure what you’re doing, but keep doing it!”
Another example is this aerospace company I was working with. It used our software, and luckily, it followed this recommendation to take data, once a week, step back, aggregate it, summarize it, and extract the information from it. The company eliminated its scrap in about a three-month period, saving tens of millions of dollars in these huge products that it was making. So, yes, data interrogation works. If companies will just step back and regularly interrogate their data, they’ll uncover meaningful information that provides immense return on their quality investment.
Find out you can achieve strategic decision-making and transformative results. Access free info now. |
Add new comment