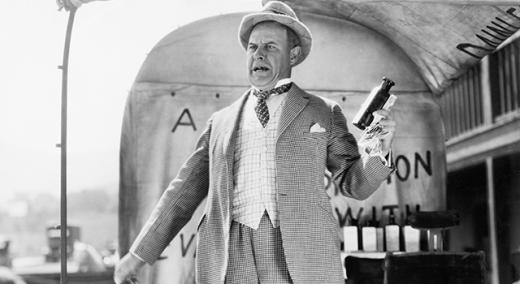
One of the most common questions about any production process is, “What is the fraction nonconforming?” Many different approaches have been used to answer this question. This article will compare the two most widely used approaches and define the essential uncertainty inherent for all of these approaches.
ADVERTISEMENT |
In order to make the following discussion concrete we will need an example. Here we shall use a collection of 100 observations obtained from a predictable process. These values are the lengths of pieces of wire with a spade connector on each end. These wires were used to connect the horn button on a steering wheel assembly. For purposes of our discussion let us assume the upper specification for this length is 113 mm.
Figure 1: Histogram of 100 wire lengths
…
Comments
So What is the fraction Nonconforming
Is there a citation for the Agresti-Coull equation? Curious about where they get the 2 and the 4.
Another nail
When stepping back and forgetting everything about statistics for a moment, it is possible to see that the usual talk about parts per million becomes meaningless. Simply by looking at a histogram, you can tell that there is just no way that enough information is present in the samples to extrapolate to anything about parts per million.
Six-Sigma is sloppy with its parts per millions (and billions!) and has not bothered to carry the burden of evidence. Instead, the burden has been reversed and left to the critics. But disproving something is much harder than making claims and less glamorous, so I think this is important work from the author.
Estimates
My critique of many Six Sigma authors is that they do not understand that any sample can only give you an estimate, and that estimates carry uncertainty. I have read (and don't ask for a citation...I read this years ago in an article) that if your process produces 6 parts per million defective, you have not reached "six sigma" quality!
In my view, a lot of this comes from rule 4 of the funnel. Jack Welch didn't know anything about statistics, but he knew how to sell Wall Street on what he thought was a great cost-cutting scheme. This did a number of things: Popularized Six Sigma in the CEO set, which created demand for Six Sigma consultants and trainers, which led many consulting firms (largely populated by accountants) to get on the bandwagon and develop programs that demanded projects that would yield $250K or more (and rejected any that did not). At GE, engineers who felt that "It's better to have a sister in a cathouse than a brother in quality control" and "a camel is a horse designed by a team" and "the quickest way to ruin something is to improve it" were suddenly forced to become Black Belts. (The quotes, by the way, are from my father, a life-long GE engineer...when I told him what my new career aspirations were after going to a Deming seminar). So now you had a lot of Black Belts who were forced to go to training that did not interest them...some of them probably had projects that didn't make the 250K cut, and so essentially pulled 16 red beads, made the bottom 10% and ended up on the street. At that time, guess which bullet in their resume made them valuable? GE Black Belt. So now you have novices being trained by neophytes. Many of them ended up as consultants or trainers...
Add to this that statistical thinking is counterintuitive and not something that humans inherently do; they have to be taught, they have to practice, they have to want to learn and to care. Most people don't, and most Americans, taught statistics for enumerative studies, couldn't begin to understand Deming or Wheeler or Nelson. As engineers and accountants, they believed in the precision of numbers and the reliability of prediction.
So, it's OK to estimate a fraction non-conforming, but you have to remember it's an estimate. I have tried very hard in recent years to never, ever give a point estimate. I have adopted an approach I learned from Heero Haqueboord: when someone asks me for "the number," I tell them, "I'm pretty sure it's going to be somewhere between x and y." When they press me for a single number they can use for planning, I repeat, "I'm pretty sure it's..." They might get angry about it, but I usually end up telling them, "Look, I could give you one number, but it will be wrong. If anyone tells you that they are certain it is THIS number, run away from them and never listen to anything they say again."
In favor of model-based estimate for a pharmaceutical process?
Operating in the pharmaceutical industry, is it not more realistic to report a model-based estimate of the process nonconforming fraction? Assume a company makes 30 or 100 batches of a pharmaceutical over a span of 5 or 10 months or years, all within-specifications (Y = 0).
Imagine what would be the reaction of a boss when informed that the nonconforming fraction of batches generated by a well-behaved predictable GMP-compliant manufacturing process could reach 13.8% and 4.6%, respectively? The Wald interval estimate and the Agresti-Coull limits are essentially based on n Bernoulli trials, in fact on a sampling experiment whereby n = 30 or n=100 batches are randomly selected from an infinite population of batches.
As we all know, data should be evaluated in their context. In reality, each batch is produced separately. Is it not preferable to adopt a simple practical approach: to collect the data, to characterize their distribution by fitting a reasonable model and then derive the nonconforming fraction? Yes! The two disadvantages of the probability model mentioned by Dr. Wheeler (arbitrary probability model and data extrapolation) still hold. But, the outcome (a smaller nonconforming fraction) will be more consistent with the reality of zero nonconforming throughout repetitive manufacturing across a large span of time.
Yes! Statistics change. Given the information one has about his process at this point, one estimates the nonconforming fraction. As of today, the process generates zero and the expected nonconforming fraction is consequently small or very small. As more data are collected, an updated nonconforming fraction wll be calculated and reported. Isn't this practical approach preferable rather than reporting a worst-case value that appears non-consistent with the present behavior of the process?
To the best of my knowledge, the probability model is commonly applied in the pharmaceutical industry.
Commonly applied =/= right
Being commonly applied is not the same thing as being right. It is certainly commonly applied in the medical device industry as well, so I'd venture that the same holds in pharma. But the whole point of this article is that this approach does not have enough data to produce an adequate level of certainty relative to the estimates. After getting 30 samples with zero failures, no one is claiming that the true failure rate is 4.6%, they are merely stating that we can't reduce the upper limit of certainty to anything below 4.6%. The true value might be 3.4 ppm, or 3.4 parts per thousand. No one can possibly know with such a limited amount of actual data. The probability model approach is just a way of lying to yourself about how precise the esitmate is. As Wheeler pointed out, there are many different probability models that you could fit and they will produce wildly different results. So why should you trust any specific one vs calling your upper limit essentially the worst case of any of the models you could possibly fit?
Great point!
"The probability model approach is just a way of lying to yourself about how precise the estimate is."
That's an excellent way to sum it up. As to using the worst case out of all the models you could fit, Wheeler wrote some articles a few years ago arguing that the normal distribution is the distribution of maximum entropy.
I'm not always successful in this, but I always try to keep in mind a paragraph from Shewhart's Statistical Method from the Viewpoint of Quality Control:
"It must, however, be kept in mind that logically there is no necessary connection between such a physical statistical state and the infinitely expansible concept of a statistical state in terms of mathematical distribution theory. There is, of course, abundant evidence of close similarity if we do not question too critically what we mean by close. What is still more important in our present discussion is that if this similarity did not exist in general, and if we were forced to choose between the formal mathematical description and the physical description, I think we should need to look for a new mathematical description instead of a new physical description because the latter is apparently what we have to work with."
reply for ANONYNOUS
The Agresti-Coull confidence interval uses the Wilson point estimate. The Wilson point estimate adds the z-score for the alpha level to both the number of successes and the number of failures. So, for a 95% interval we add 1.96 successes and 1.96 failures to the counts. Since we are dealing with counts we typiclaly round these off to 2 successes and 2 failures. For a 90% Agresti Coull interval the Wilson point estimate would add 1.65 failures and 1.65 successes, etc. The Wilson point estimtes were used in yet another formula for a confidence interval, done in the first half of the 20th Century. In 2003 Agresti and Coull did an extensive survey of all the ways of obtaining a confidence interval for a proportion, analyzed how they worked in practice, and came up with their synthesis that is now the preferred method for such a confidence interval.
Citation
Here's a citation for you, anonymous: Agresti, A., & Coull, B. A. (1998). Approximate is better than “exact” for interval estimation of binomial proportions. The American Statistician, 52(2), 119-126.
Don, I have to ask, though...why n+4 if we're adding 2 to the failures and 2 to the successes? I understand Y+2, but why n+4? To adjust when you take the square root?
Add new comment