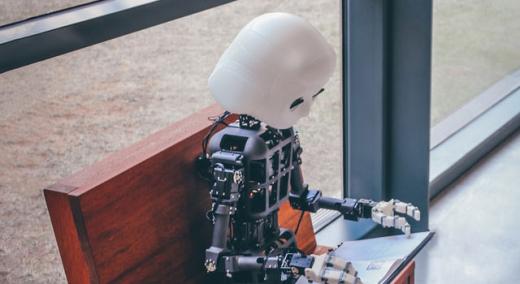
(MIT: Cambridge, Massachusetts)--For workers who use machine-learning models to help them make decisions, knowing when to trust a model’s predictions is not always an easy task, especially since these models are often so complex that their inner workings remain a mystery.
ADVERTISEMENT |
Users sometimes employ a technique, known as selective regression, in which the model estimates its confidence level for each prediction and will reject predictions when its confidence is too low. Then a human can examine those cases, gather additional information, and make a decision about each one manually.
But while selective regression has been shown to improve the overall performance of a model, researchers at MIT and the MIT-IBM Watson AI Lab have discovered that the technique can have the opposite effect for underrepresented groups of people in a dataset. As the model’s confidence increases with selective regression, its chance of making the right prediction also increases, but this does not always happen for all subgroups.
…
Add new comment