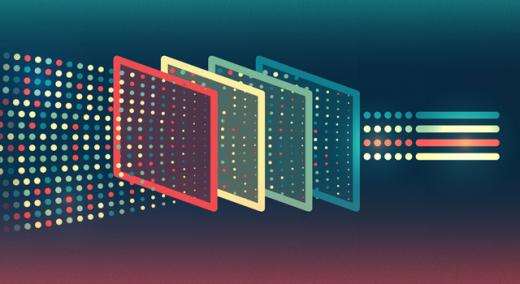
When machine-learning models are deployed in real-world situations, perhaps to flag potential disease in X-rays for a radiologist to review, human users need to know when to trust the model’s predictions.
ADVERTISEMENT |
But machine-learning models are so large and complex that even the scientists who design them don’t understand exactly how the models make predictions. So, they create techniques known as saliency methods that seek to explain model behavior.
With new methods being released all the time, researchers from MIT and IBM Research created a tool to help users choose the best saliency method for their particular task. They developed saliency cards, which provide standardized documentation of how a method operates, including its strengths and weaknesses and explanations to help users interpret it correctly.
…
Add new comment