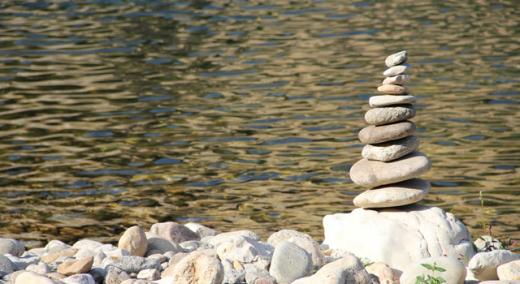
Credit: Mathieu Turle on Unsplash
The success run theorem is one of the most common statistical rationales for sample sizes used for attribute data.
ADVERTISEMENT |
It goes in the form of:
Having zero failures out of 22 samples, we can be 90% confident that the process is at least 90% reliable (or at least 90% of the population is conforming).
Or:
Having zero failures out of 59 samples, we can be 95% confident that the process is at least 95% reliable (or at least of 95% of the population is conforming).
The formula for the success run theorem is given as:
n = ln(1 – C)/ ln(R), where n is the sample size, nl is the natural logarithm, C is the confidence level, and R is reliability.
The derivation is straightforward and we can use the multiplication rule of probability to derive it. Let’s assume that we have a lot of infinite size, and we are testing random samples out of the lot. The infinite size of the lot ensures independence of the samples. If the lot was finite and small, then the probability of finding good (i.e., conforming) or bad (nonconforming) parts will change from sample to sample if we’re not replacing the tested sample back into the lot.
…
Add new comment