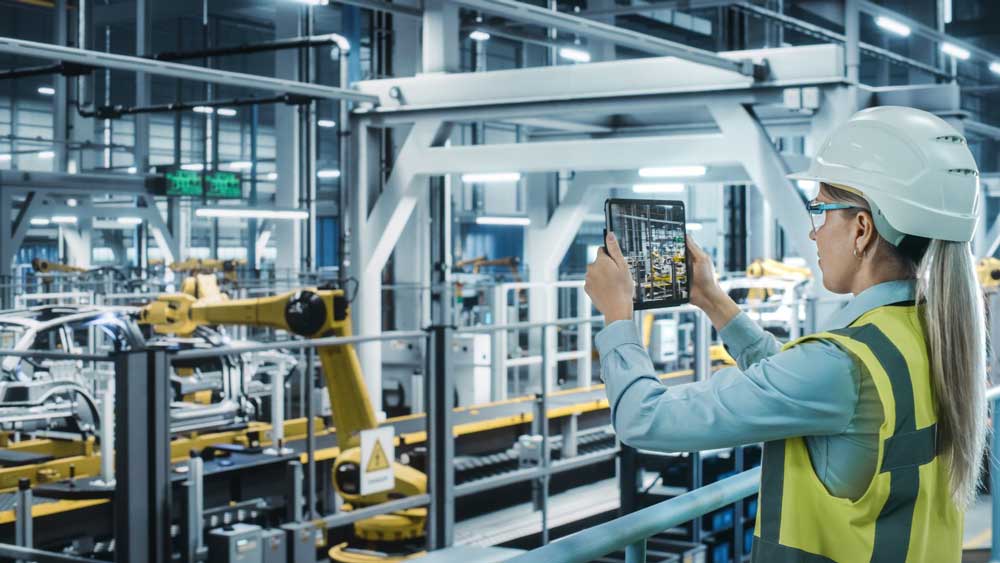
Welcome knowledge seeker! Do you feel dazzled and awed by the great potential of artificial intelligence (AI)? Perhaps hesitant or lost when terms like convolution, deep learning, or autoencoder are thrown around? Well, fear not, for you’ve come to just the right place. You don’t need to be a computer wizard or a super-genius to understand how using AI can affect your system and, more importantly, whether using it is worth the investment.
ADVERTISEMENT |
This is the first of a multipart series aimed at helping you practically evaluate each step of investing in and using an AI-enhanced system or tool in an industrial application. We’ll address the fundamentals of what industrial AI (IAI) is and how it differs from your everyday AI. We’ll discuss its development, considerations for training, and concepts for practical evaluation in a live or test-grade environment. Although we won’t be covering everything you’ll need to know, you’ll get a good sense of the what, when, and why of assessing your IAI.
…
Add new comment