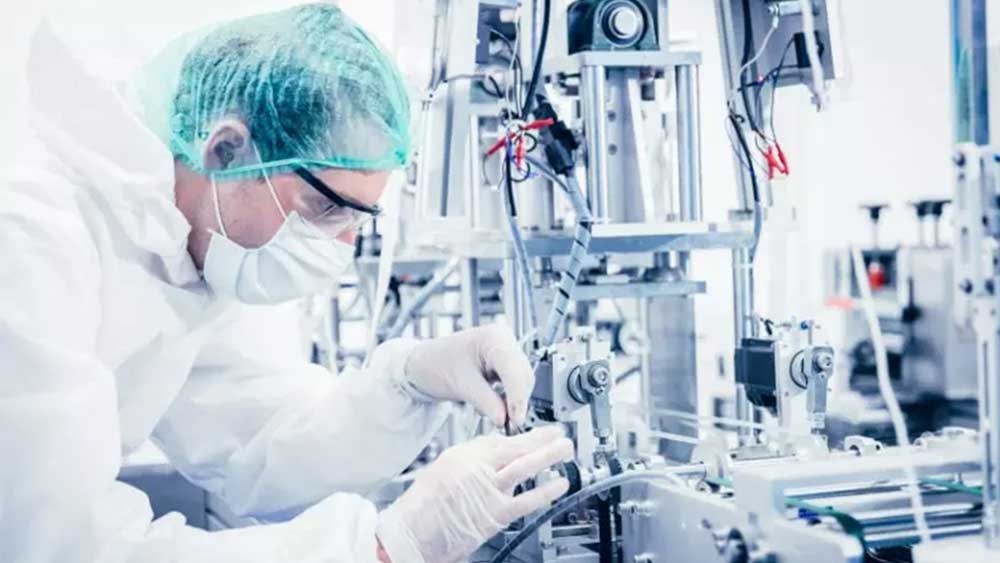
In April 2018, the U.S. Food and Drug Administration (FDA) approved the first artificial intelligence-powered diagnostic system, a software program used to detect diabetes-related vision loss.
ADVERTISEMENT |
Since then, the industry has seen explosive growth of AI in medical device manufacturing, which is transforming every step in the process from design to production to postmarket surveillance.
Where is the industry headed, and where can we expect to see the greatest effects? In this article, we look at why and how AI is revolutionizing medical device manufacturing, and what the FDA has signaled about the compliance issues that lie ahead.
The rise of AI in medical device manufacturing
The market for AI in medical devices is experiencing massive growth and is expected to surge from $15 billion in 2023 to a whopping $97 billion by 2028.
…
Add new comment