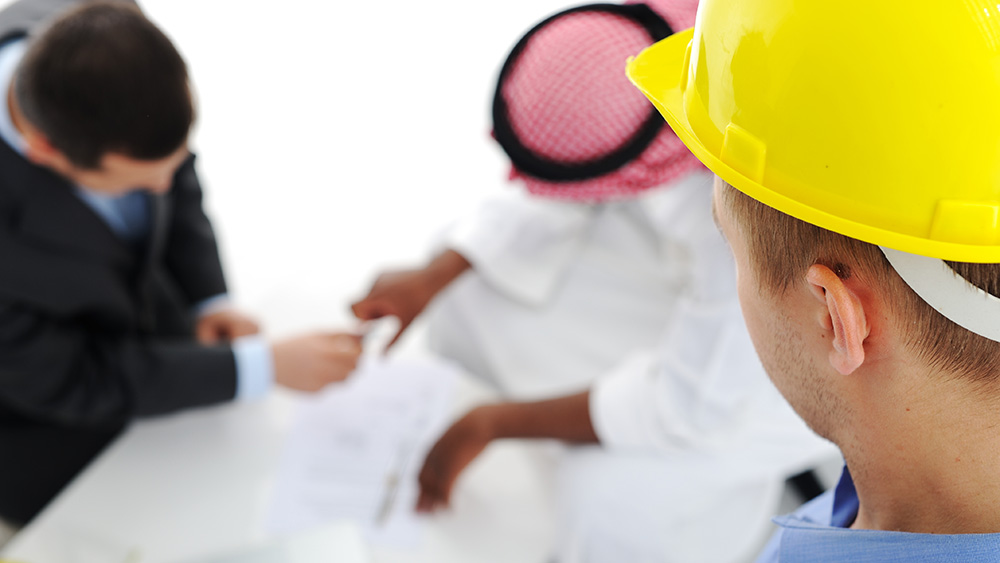
Photo from Storyblocks.
Many marketing professionals are drawn to their field because they consider themselves more right-brained (creative) than left-brained (analytical). It’s true that success in marketing often requires imagination, creativity, and an emotional appeal to the target audience. In fact, many college students choose marketing as their major due to the perception that it is a less rigorous discipline than fields like finance, physics, or engineering. In the workplace, marketing has been seen as a profession driven more by intuition and creative flair than analytics and data. However, with the rise of digital tools and the increased prominence of data-driven strategies, intuition and creativity alone are insufficient for marketing pros.
ADVERTISEMENT |
Today, marketing has evolved into a field where analytics and data management are just as important as creativity and imagination. Marketers, once stereotyped as being “soft on data,” must become proficient in data analysis. In fact, many marketing campaigns now rely heavily on the insights provided by data analytics to optimize performance and improve outcomes.
…
Comments
Possible further data analysis
I had the opportunity to listen to Alfredo present at a recent ASQ meeting and he covered this topic. When he asked for questions, I posed the following - "While this analysis certainly provides more insight than the simple % calculation, might there be some additional insights to see if the <4 ratings are skewed closer to 1 or 3?" Perhaps not, but maybe the seemingly "arbitrary" choice of 3 being "bad" needs to be investigated. As mentioned in the article, there may be outliers that are skewing the data. The point is, using statistical techniques on "soft" marketing data, can lead to better understanding of what is "really" going on.
Add new comment