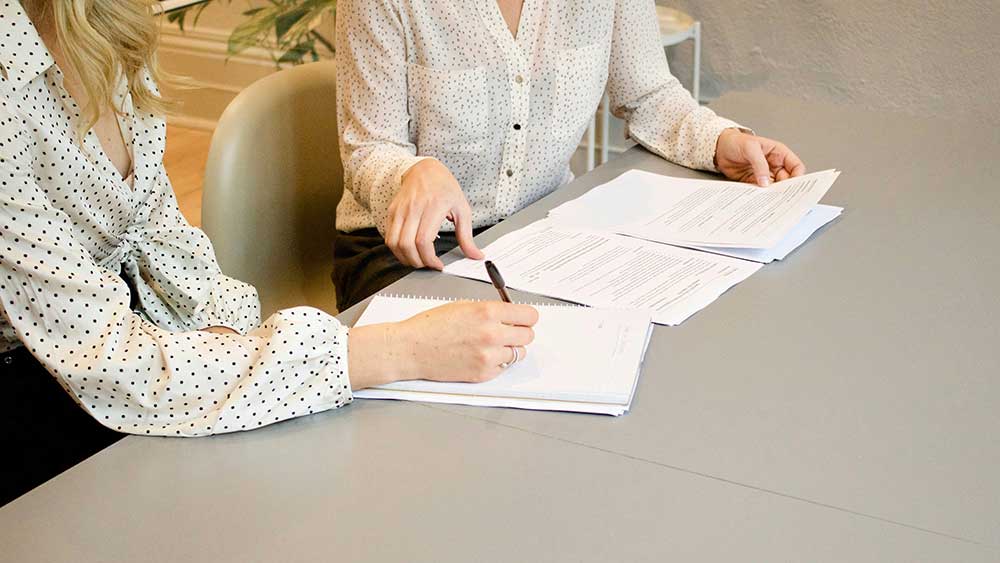
Photo by Gabrielle Henderson on Unsplash
In last month’s article, “ANOVA and the Process Behavior Chart,” we saw how both techniques use the same basic comparison to answer completely different questions. Here, we’ll look at a case history where both techniques were used.
ADVERTISEMENT |
A physical property of a mass-produced item was important to its functionality. The production of these items involved three steps: batches of compound were mixed, molded into parts, and coated. In an effort to maximize a product characteristic, an experiment involving three production variables was carried out. Factor A was studied at two levels; Factor B was studied at three levels; and Factor C was studied at five levels. So the fully crossed study required 30 experimental runs. For each run, a sample of 40 parts was selected from the output and each part was measured. Thus the manufacturer had 30 treatments with 40 observations per treatment for a total of 1,200 observations.
…
Add new comment