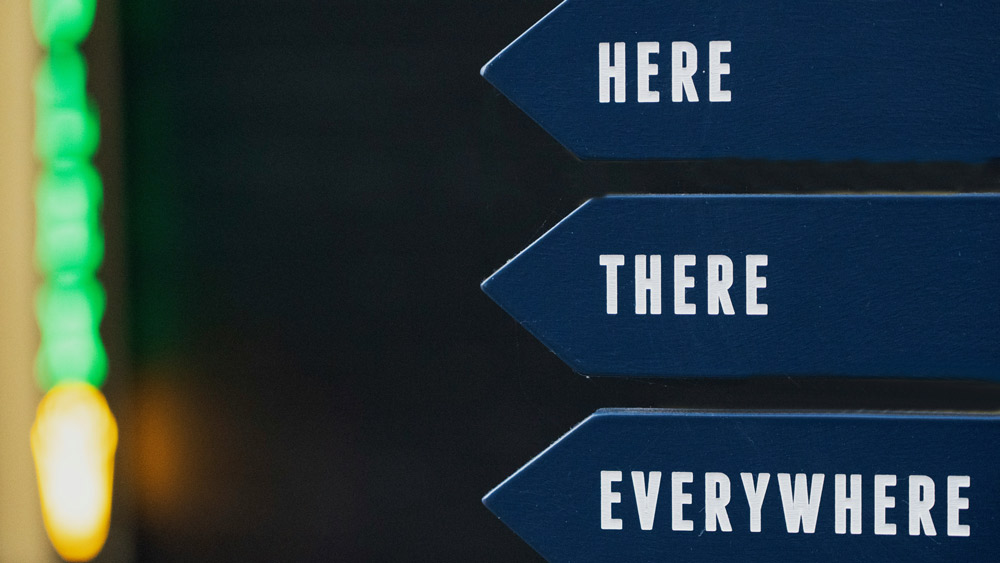
The far-reaching accessibility and innovations of AI-driven maintenance make operations safer, faster, and better. Photo by Nick Fewings on Unsplash
Unplanned equipment downtime costs manufacturers an estimated $50 billion annually, according to a study by Deloitte. It is estimated that unplanned downtime costs more than $100,000 per hour. Traditional maintenance approaches—whether reactive or scheduled—are not sufficient to address these inefficiencies. Artificial intelligence (AI) offers a transformative solution to equipment maintenance: predictive maintenance.
ADVERTISEMENT |
By leveraging AI and IoT (internet of things) technologies, predictive maintenance enables manufacturers to monitor equipment health, predict failures before they occur, and optimize repair schedules. This game-changing approach not only minimizes downtime and costs but also enhances safety, sustainability, and operational efficiency.
…
Comments
We propose starting with an economically viable step
To transition to predictive diagnostics in the maintenance of critical equipment, we propose starting with an economically viable step, namely, diagnosing using Statistical Process Control (SPC) methods.
Advantages:
Minimal data volume requirements. Monitoring data and diagnosing using SPC methods require incomparably less data than diagnosing with machine learning (ML) methods. For diagnostic and forecasting purposes, it does not matter whether the data represents 100% of the data series or just a part of it; however, the data must be organized rationally and with consideration for temporal distribution.
Data collected manually will suffice. As a result, there are minimal requirements for the computational power of the equipment and the storage of historical data (continuous data collection is not required).
Add new comment