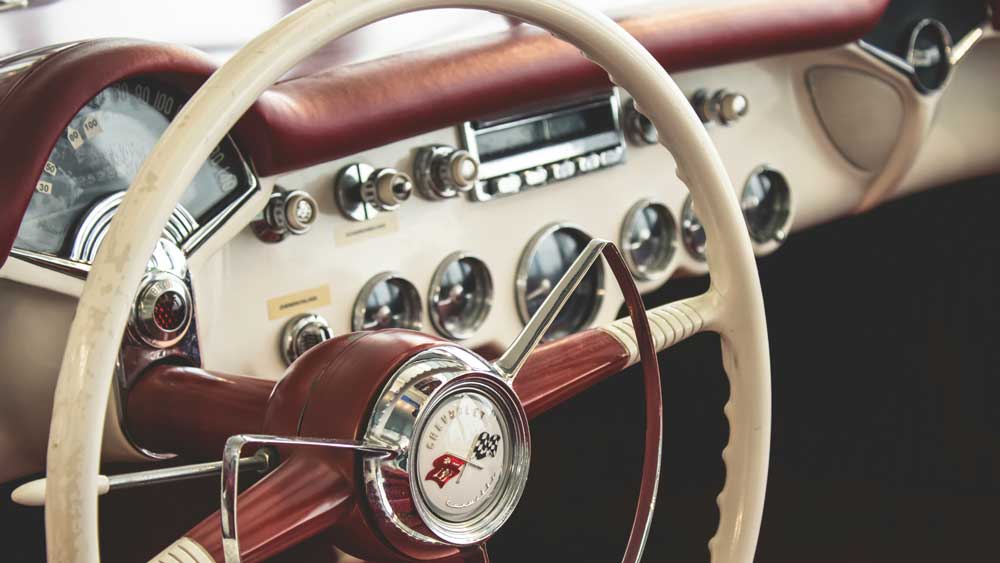
Photo by Niklas Garnholz on Unsplash
Managers are commonly fed a diet of report-card data. These data have usually been aggregated into summaries, averages, and totals to characterize the big picture. As useful as such summaries can be, they can also be an obstacle to an effective analysis. Here, we’ll learn how to avoid this obstacle.
ADVERTISEMENT |
Our example will come from a plant producing steering wheels. At the end of the production line, the inspectors would either pack the wheels for shipment to the assembly plant or place them on a line going to the rework department. Each day, Andy would visit the inspection station to conduct a 60-piece audit. When one of the inspectors rejected a wheel, she would tell Andy why she had rejected that wheel. Andy would add a tick mark to his sheet, and after 60 wheels had passed he would return to the quality office across the street and record the data.
The chart Andy produced for management consumption was an np-chart showing the total number of wheels rejected for each audit. This report-card chart is shown in Figure 1.
…
Comments
Go to the Gemba
Good article Don
This emphasizes the need to go where the action is (Gemba) and understanding how the data is collected to solve the problems. The best Managers also go to the Gemba.
Great advice
Yes, we need to know how good the stuff is that we make, but knowing this doesn’t - as well explained and illustrated here - necessarily give any clues as to how to make things better. Find the context in and around your data, use this context, disaggregate the data, and measure upstream: Great advice.
Thanks to Dr. Wheeler for another year of excellent monthly columns in QD.
Summarized data has no nutritional value
To find the "hidden low-hanging fruit" I encourage people to go on a "raw data" diet, just as this article suggests.
Where's your raw data about defects, mistakes and errors?
With enviable consistency in the quality of articles
With enviable consistency in the quality of articles, along with specific examples and recommendations, Dr. DONALD J. WHEELER is a treasure trove of true knowledge. It is not surprising that Dr. W. E. Deming particularly valued Dr. Wheeler's contribution to the methodology of using Shewhart control charts.
Add new comment