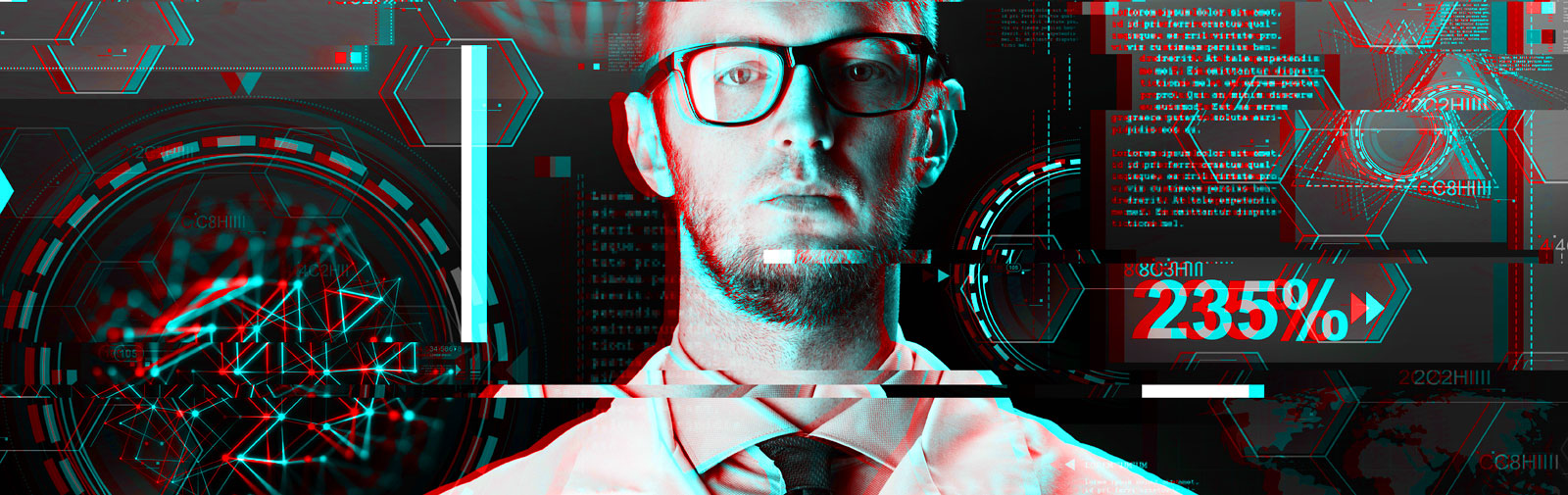
Blame it on Moore’s law. We live in a digital Pangaea, a world of borderless data driven by technology, and the speed and density with which data can be transmitted and handled. It’s a world in which data-driven decisions cause daily fluctuations in markets and supply chains. Data come at us so fast that there is almost no way business leaders can keep abreast of changing supply chains and customer preferences, not to mention react to them.
Operating any kind of manufacturing today requires agility and the means to turn the flood of largely meaningless ones and zeros into something useful. The old ways of treating data as nothing more than digital paper won’t cut it in the “new normal.” We need to reimagine how we view quality.
Disaster, war, pestilence... and customers
During the last few years alone, we have seen supply chains brought down by natural disasters, and a trade war with China that has caused purchasers and vendors alike to scramble for new sources or customers. Covid-19 and the worldwide-shutdown have shown weaknesses in existing supply-chain logistics that nobody could have imagined. Who would have anticipated dumping dairy products onto the ground, or having to slaughter and dispose of livestock even while grocery shelves had no dairy or meat? All for the lack of end-to-end process visibility and the data that drives it.
Consumer preferences and shopping habits literally—not metaphorically—change overnight. Younger buyers want greener products, or those that promote fair trade practices. Nielson reports that 75 percent of millennials are willing to pay up for sustainable products. For manufacturers, this often means changing raw materials or raw-material providers, which can lead to process changes and changes in quality controls.
Quality reimagined
For a single-site manufacturer with relatively few employees or vendors, adapting to these changes is a headache but at least manageable. The problem grows exponentially more complex as you add employees, sites, vendors, SKUs, and processes.
Anytime an engineering change comes in, even for something as simple as a single part, that information must be communicated, from purchasing all the way through to manufacturing and into the warehouse... in some cases as far as downstream customers who rely on your assembly for a larger part.
If a part change requires a manufacturing process change, it must be communicated to everyone involved in the process. In the case of those companies that track internal or customer rejects, a return data channel—feedback—must also be in place to let manufacturers know about problems and to give them some way to correlate nonconforming products to parts or process changes.
Standing in the middle of all this is the quality department. For years quality professionals have been hampered by siloed data and the inability to access and react to risk across the company. And yet, their job relies on the ability to see quality metrics of every stripe, not just inspection failures, but customer complaints, engineering change orders, returns, even material usage.
To break down the silos and let the quality function do its job, quality needs to be reimagined. Not the way quality analysis is done—Walter Shewhart’s tools are as relevant today as they were 90 years ago—but in how quality data are collected and presented. Today’s quality professionals must be able to identify problems in real time and react to them in real time.
A reimagined quality stands on three pillars: data, which are the drivers; decisions, based on those data; and direction, or the control mechanisms that you put in place because of the data.
Data
We already have data. Too much data, some would argue. In a modern manufacturing facility, you have data spewing from production equipment, much of which go unused. You have data from your alphabet soup of ERP, QMS, EMS, MAP, MES, PIM, and CRM systems, most of which also go unused. You might also have data from IIOT devices reporting on physical plant metrics.
Even if you have a statistical process control (SPC) system that automatically captures data and produces beautiful control charts, how are they used? If they are just printed out and posted on a wall with 100 other control charts, they become nothing more than visual noise. It’s actionable data if they are examined, but how many people will sort through the clutter?
While data are good, good data are better. For data to be useful they must be more than just present; they must be relevant to a company’s cost, value, and risk needs. And most important, they must rise above the noise and be useful to everyone who touches them.
Good data must be:
Complete: You must have all the data you need to perform an analysis, without muddying the waters with data you don’t need. For instance, if you are analyzing machine up-time, you would, at a minimum, need data relating to the machine ID, location, time stamps, and maybe the operators. You need to question whether any data beyond that help you with your analysis or just fog your view.
Consistent: Nothing eats up more time in data analysis than trying to align what should be similar data that come from different sources. As much as possible, all data for a certain analysis should be collected in the same way and presented in the same way and in the same order, no matter the source. How useful is it to compare process data from Line A collected every minute, with the same process data from Line B collected once per day?
Efficient: This means that you have access to any data, on any device, at any time. No silos, physical or temporal. Data are useless if you can’t quickly and efficiently access them. If data are hard to access, they won’t get used. Efficient analysis applies here as well. Too many data—or irrelevant data—that have to be excessively manipulated, even on a computer, eats up time.
Widely available: Related to data efficiency is availability. Do the right people have access to the data they need, when they need it? Can they access it remotely? If your data are siloed between departments or facilities, the entire organization suffers. Think how important customer relationship management (CRM) data might be to the quality team.
Centralized: There needs to be a single source of truth, a single place where data are securely stored. This could be an in-house server or cloud server, but the important point is that all parties are able to access the data from anywhere and at any time. Organizations with multiple sites should be able to instantly see what is happening from an enterprise perspective, along with the ability to drill down into sites, and processes within sites.
Real time: Data are captured “as it happens” and can be made available for immediate feedback, analysis, and control as well as monitoring trends in real time.
In a truly digital environment, technology takes care of routing, filtering, and analyzing data to specific rules without human intervention. If a process starts to run out of control, based on whatever rules you have assigned, the software can immediately notify the appropriate person and show him only the data that make sense for a given event. The goal is not only real-time access, but also less clutter and high visibility.
Quality data: All of the above can be said to fall under the umbrella of data quality. Rupa Mahanti, in her article “Critical Data Elements and Data Quality,” writes that “Data are considered of high quality if they are fit for their intended use. In other words, data quality can be defined as an evaluation of whether those data serve a purpose in a given context.”
Some examples of data-quality dimensions that Mahanti lists are completeness (i.e., whether values are present or absent), uniqueness (extent to which the data relating to an entity are not duplicated), and accuracy (the data values’ closeness to reality).
Decisions
Quality data essentially have two lives. The first is how we immediately use it. A control chart that gives us feedback on a process so that we can spot process problems is an example of data’s first life.
Data’s second life is about strategic decision making. What long-term trends do they show us? How do we correlate customer complaints to process changes? Why does one site perform differently than another? Can we look at contextual data from each site and correlate that to differences in performance? There is so much potential knowledge to be extracted from data’s afterlife that is largely overlooked, mostly because the data are hidden away in the electronic equivalent of a file cabinet in a dusty storeroom: unseen and unknown.
In either case, we need to rethink how data should be presented to us. We don’t really need to see several dozen control charts. We only need to be informed if a process is out of control. Let the computer do the work, while giving the user to ability to drill down if needed.
In order to make quick decisions, all stakeholders need easy access to the data no matter where they are or what computing device they are using. Intuitive, easy-to-interpret data must be available in real-time anywhere, any time.
Direction
We not only have to collect data, we also have to treat them correctly, focusing on exceptions and rules, and presenting the data in a way that leads to better control of a process. Cluttered dashboards that show everything instead of just what needs to be seen aren’t useful.
The implications of this approach are huge. The ability to understand and react to a process no longer needs to reside with quality professionals or those who can read a control chart. Any employee can learn to read a dashboard that tells her only what she needs to know. Her own process knowledge can then help her to identify the source of the problem. Any employee—from shift supervisor, to machine operator, to supply chain exec, to plant lead—can know what decisions need to be made and make the right decisions. Every employee becomes a knowledge worker.
Changing the way we use quality tools and present quality data will lead to the democratization of the quality function.
The new normal
Now that we are on the—hopefully—waning end of the Covid-19 pandemic, the phrase “new normal” is used a lot when it comes to the workplace. One thing that has become abundantly clear is that while the issue of data collection, analysis, and remote access has been on everyone’s back burner for years, the latest crisis has brought these issues to the fore.
Now is the time to look at the data you most likely already have, and learn how to leverage them. The task of bringing data and data analysis to the forefront may seem a bit daunting, but it’s not impossible. Yes, there are issues to overcome. The biggest is probably manpower. Who is going to take on the responsibility for not only deciding what data we need, but also how to handle it? What skills are needed? Will we need to hire another person just to understand how this works? And, of course, how are we going to pay for it? How do we calculate our return on investment?
But as recent events have shown, this must be a priority. For some companies, looking at the past three months, the ROI is glaring. There is no way manufacturing of the future cannot put proper data collection and analysis at the top of its list. Building flexible, capable, and resilient foundations for the future depend on tackling these issues.
Comments
Using Our Data - - Book Reviewed
Yes - - Yes, Using Our Data is a value of accomplishing studies & projects resulting in data, findings, discoveries, & more we can use. I recently completed a review of the new book "Living Documentation - - Continious Knowledge Sharing By Design" (author - Cyrille Martraire - - publisher - Addison-Wesley) for the ASQ Quality Dijest magazine. The book definitely fits into thinking about using our data, effectively managing our data for our use, & building knowledge as we move forward in the use of our data.
Add new comment