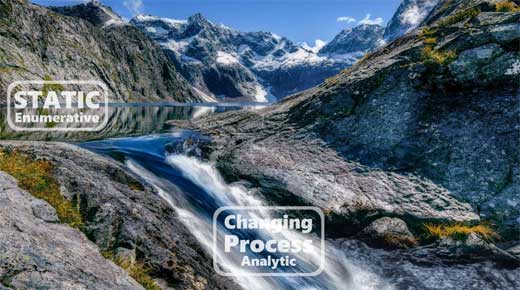
Quality is related to processes. A process is “a series of actions or steps taken in order to achieve a particular end.” It doesn’t matter whether the process is the handling of invoices, customers in a bank, the manufacture or assembly of parts, insurance claims, the sick passing through a hospital, or any one of thousands of other examples. A process involves movement and action in a sequential fashion.
ADVERTISEMENT |
Every quality professional is concerned about the improvement of processes. By making processes better, we get less waste, lower costs, and happier customers.
![]() Photo used with permission of Samuel Ferrara. |
The image above depicts two opposed states: a dynamic, changing state and a static state. The lake is static, unchanging. We might take temperature measurements in the lake at different depths and come back tomorrow to find no difference. We might take samples of the lake water to compare with other lakes at a later date when we travelled to them.
…
Comments
Six Sigma - Prediction Failure
Six Sigma focusses on enumerative tools. M Harry, the creator of Six Sigma, was asked a similar question to that of this article "Could you explain the best way to predict the outcome of a process in the future?" Harry's answer showed complete ignorance of analytic methods and the meaning of a predictable process: "Reference the quality literature on statistical process control, also known as “SPC.” There are many excellent books on the subject. Process improvement and optimization is often accomplished by way of statistically designed experiments, or “DOE” as it is known."
A Six Sigma process with its +/-1.5 sigma shift is wildly out of control and is hence unpredictable. It cannot produce good quality product/service in the future, no matter where specification limits are set.
Further...
I would agree that most of the Six Sigma world has left its quality roots behind, chasing cost cutting and forgetting Deming, SPC, the Taguchi Loss Function and its implications (which concepts are what the whole idea is, after all, based on). I believe that one reason for the emphasis on enumerative study methods is because they are, unfortunately, what are taught in most statistics courses at the high school and university level. Hopefully, some of the research in the list of papers I provided above will shake the enumerative statistics world enough to effect some change.
There are very few textbooks outside the sort of "deep quality" realm that even discuss analytic or enumerative studies. To advance the notion of analytic studies--and to see it survive us--what is probably needed is some sort of analytic statistics society with enough academic credibility to publish its own journal.
As far as the 1.5-sigma shift goes, it doesn't exist...despite it's presence in international standards as a "convention." In the real world, assuming some reasonable attempt at statistical process control, a process whose data display a sustained but undetected 1.5 sigma shift CANNOT exist. Platitudes such as "Shift Happens" are cute but do not reflect the real world.
Analytics Society
Rip - I suspect that you on to something that might increase the credibliity and visibility of analytic studies. There are many of us out there trying valiantly to change things. In my own organization I am continually fighting against the resurgence of enumerative studies and what I call "statistical alchemy' (FMEA/RPN, Cpk/Ppk, AIAG Gage R&R, etc.). Even though the organization ahs demonstrated great success with analytic studies the seduction of not having to think (just take a pile of numbers and throw them into some software) is so powerful.
I will be presenting at the ASQ Lean and Six Sigma conference in March and the Worl Quality conference in May...fighting the good fight. perhaps I'll see you at one of them?
Analytics Society
I'm just afraid that the window is closing. It probably would have taken people of the stature of Myron Tribus or David Kerridge to pull it off...
Hypothesis Testing
Are you saying Quality professionals have no use for Hypothesis Testing? I understand Hypothesis Tests assume static states. What if I am trying to reduce variation in a molding process (process improvement) and want to compare products coming from two cavities. Of course to compare means from the 2 cavities the mean would have to be a useful statistic (implying a stable process characterized by a single distribution). I do not see why you are bashing the fact that quality professionals learn statistical methods for making valid comparisons while taking into account the inherent variability in the data.
Molding Cavity Variation
I would refer the reader to Wheeler and Chambers' Understanding Statistical Process Control for an excellent example of the use of control charts to solve the problem you have posed. Through the use of rational subgrouping, the manager of that process was able to look at hour-to-hour, cycle-to-cycle and cavity-to-cavity variation. Cavity-to-cavity variation turned out to be the greatest driver of variation. The manager used the charts (and the process knowledge of his molding machine operators) to reduce between-cavity variation, and then monitor it using a dynamic and very sensitive method.
Casting
Thanks Rip. Dr Wheeler also discusses such casting here: https://www.qualitydigest.com/inside/quality-insider-column/060115-rati… and in "Advanced Topics in SPC" "Rational Subgrouping" pages 143 to 157. These books are essential for anyone serious about quality.
As you can see from Dr Wheeler's examples and the example in my article, hypothesis testing throws away key data that can be used to help analyse your process. There is absolutely nothing wrong with hypothesis testing when used correctly. Hypothesis testing is fine for static, non process related situations.
Enumerative studies were promoted in Six Sigma courses. However the enumerative tools of Six Sigma are inappropriate for analyzing processes. Six Sigma was developed by a psychologist. It is hardly surprising that it focuses on the enumerative tools that are far more appropriate for lab rats in a psychology laboratory, than for process improvement.
More on "static" processes
Further to above points, what proportion of many man made processes display stable behaviour over time (i.e. static)?
I think a valid concern is the use of hypothesis tests without a prior check on whether the data being used in the test are “in control” or not.
When the data are not stable the use of the hypothesis tests is questionable.
When the data are stable the control chart can often be used to answer the question to be answered through a hypothesis test.
Great point, Scott
I don't know the proportion of man-made processes that are stable; you can't know until you look. You make a great point about stability and hypothesis tests, though. This was the reason Shewhart developed control charts - to test whether the data exhibited a reasonable degree of statistical control. If they do, then distributional assumptions could be made. Without the evidence of homogeneity we get from the charts, we have no evidence for any particular distributional model. If the process is stable, then you are correct; processes can be compared handily using those charts...no need for t-tests, z-tests, ANOVA.
Thanks, Rip
I had an example this afternoon.
Three subgroups of n=3: No signal on the range chart (or S chart). No signal on the average chart.
We ran the ANOVA and looked at the table of values: No signal. I asked my colleague which was easier to understand. What do you think the answer was?
My guess
Just a guess, but if your colleague was someone who grew up in the enumerative world, I'm guessing they thought they understood the high p-value in the ANOVA better. My other guess is that they don't really understand that p-value (and what it DOESN'T say). I've never understood why a simple visualization would not be preferable, but it might be as Wheeler points out, "Some people are afraid of simplicity, because complexity looks profound even if it's absurd."
Dr. Deming did a series on the difference between analytic and enumerative studies for Ford engineers in the early '80s. At one point he lamented that he could not get engineers to use two simple tools: a piece of paper and a pencil. ("They will be damned before they do"). He then drew a plot on the newsprint: Two high points followed by a low point, two high points followed by a low point - repeated a few times. He said, three shifts...two are high, the third is low. There is clearly a difference. No need for any advanced mathematics...just get to work finding out where the difference comes from!
Of course, one engineer asked, "But wouldn't you do a hypothesis test, just to verify the difference?"
Deming thundered, "Why? Why ruin it...waste time? You can see there's a difference, get to work!"
Love it
Love it Rip. What a wonderful reply!
Thanks, Rip (again!)
My mistake: I did a poor job of saying the colleague was happier with the average and range chart, i.e. the easy to interpret picture over the table of statistics (the ANOVA table). Nonetheless, and I had an example today, the simplicity of the average and range chart somehow seems to suggest something should replace it.
Thanks for the response. Great. I guess Dr. Deming had a way of pulling such things off in a way that few others, or nobody, could do.
Really glad to hear it, Scott!
I'm glad to hear that your colleague "got it" right away. I'm just too jaded, sometimes...
On the other hand, you might be happy to hear that I have managed to get a university to let me start teaching using one of Wheeler's texts in a course about using data in decision-making, and in a basic business stats class. So there is some (if not glaring) light in the fight for analytic studies.
Absolutely
The problem, I think, is in the education system. It is very difficult to find a stats class, or a "business statistics" class that teaches even the tools of analytic studies (much less that there are different types of studies). Those that do often do it badly (e.g., they might teach you to construct a control chart using the mean and 3 standard deviations above and below the mean for control limits).
Dear Rip, Could you
Dear Rip,
Could you elaborate more about drawing of control chart.
No need for hypothesis tests?
I would say that you don't need hypothesis tests for comparing two processes ro process streams. In my 35 yers of solving hundreads if not thousands of complex (physics based) problems, certainly executing thousands of process characterizations (emperical models that characterize teh design space of a set of inputs to critical outputs), capabilty studys, Measurement Systems analyses, V&V test plans I have never calculated a p value or performed any tests of statistical significance. If the study is designed appropriately the visual evidence available in a properly design graph will display all of the evidence you need. On teh other hand, I've seen hundreds of statistical tests that resulted in a p value less .05 and when I looked at the study design and graphed the resutl it was clear that the 'statistical significance had no practical importance or was simply incorrect becuase the process itself was non homogenous. Many statisticians - not just Deming or Wheeler - have demonstrated this.
A great paper to introduce yourself to the enumerative vs analytic study question is Deming's seminal paper: “On Probability as a Basis for Action”, American Statistician, November 1975, Vol. 29, No. 4, pp. 146-152, available for free at: https://www.deming.org/media/pdf/145.pdf
A great paper to introduce yourself to critical questioning of the usefulness of the 'hypothesis test' is "The Null Ritual - What you always wanted to know about significance testing but were afraid to ask" by Gerd Gigerenzer, Stefan Krauss, and Oliver Vitouch. Published in: D. Kaplan (Ed.). (2004). The Sage handbook of quantitative methodology for the social sciences (pp. 391–408). Th ousand Oaks, CA: Sage. © 2004 Sage Publications. Available for free at http://library.mpib-berlin.mpg.de/ft/gg/gg_null_2004.pdf
Some other papers you might find interesting:
“The Insignificance of Statistical Significance Testing”, Johnson, Douglas H., Journal of Wildlife Management, Vol. 63, Issue 3, pp. 763-772, 1999 http://www.ecologia.ufrgs.br/~adrimelo/lm/apostilas/critic_to_p-value.pdf
“The Case Against Statistical Significance Testing”, Carver, Ronald P., Harvard Educational Review, Vol 48, Issue 3, pp 378-399, 1978 http://healthyinfluence.com/wordpress/wp-content/uploads/2015/04/Carver-SSD-1978.pdf
“What Statistical Significance Testing Is and What It Is Not”, Shaver, James P., Journal of Experimental Education, No.61, pp. 293-316, 1993
Hypothesis testing
Hypothesis testing has its place, certainly in designed experiments, but in those you are dealing with experimental data (although recent literature reflected in some of the papers listed below suggests that hypothesis testing is not as universally useful as some would like us to believe). However, in recent years it has become commonplace for trainers to recommend using a t-test on before/after data to see whether an improvement action made a statistically significant difference in performance. This would be a waste of time at best, and could yield misleading results (as Dr. Burns and Dr. Wheeler point out above). If you had a stable baseline before your improvement, the process behavior chart will show you whether your change made a difference. If you made a difference, you induced an assignable cause and will see signals indicating it. You don't need a t-test.
Your best bet is to understand the difference between enumerative studies and analytic studies (if you are involved in process improvement, you are mostly involved in the latter), and use theory and methods appropriate to your study.
Other papers:
Moonesinghe, R., Khoury, M. J., & Janssens, A. C. J. (2007). Most published research findings are false—but a little replication goes a long way. PLoS medicine, 4(2), e28. doi:10.1371/journal.pmed.0040028
Tramifow, D., & Marks, M. (2015). Editorial. Basic and Applied Social Psychology, 38, (1), 1-2. doi:10.1080/01973533.2015.1012991
Trafimow, D., & Earp, B. D. (2017). Null hypothesis significance testing and Type I error: The domain problem. New Ideas in Psychology, 45, 19-27. doi:10.1016/j.newideapsych.2017.01.002
Gelman, A. (2015). Working through some issues. Significance, 12(3), 33-35. doi:10.1111/j.1740-9713.2015.00828.x
Ioannidis, J. P. (2005). Why most published research findings are false. PLoS medicine, 2(8), e124. doi:10.1371/journal.pmed.0020124
Nuzzo, R. (2014). Statistical errors. Nature, 506, 150-152.
Always Thoughtful, Useful Wisdom from Dr. Burns!
This one is another keeper!
Thank you for your kind words
Thank you for your kind words Kay.
Chi-Square
With Dr Wheeler's permission, I would like to post his wonderful email response to me, to this article on Chi Square Analysis, on the ASQ forum. The article is from a company attempting to sell enumerative tools for process improvement goo.gl/ToUJu7 How many people buy such statistical software and start pressing buttons without any clue as to what they are doing, or any understanding of the difference between enumerative and analytic methods?
"
If you have proportions for three or more conditions, and if you are willing to assume that each of the areas of opportunity represents a single universe, then you may compare the conditions by using an approximate procedure known as the chi-square contingency test.
The assumption about each of the areas of opportunity representing a single universe is simply the generalized version of the binomial assumption that all of the items (in any one condition) have the same chance of possessing the attribute being counted.
For example, the counts of units failing the on-line test for each of three shifts are:
Day shift, 85 out of 955;
Swing shift, 46 out of 940;
Night shift, 39 out of 947.
This results in a 3 by 2 contingency table, having 2 degrees of freedom. The Chi-square statistic is 22.3, and the 95th percentile is 5.99, so we can say the three shifts HAD different proportions of units that failed the on-line test.
But what does this mean? Should the three shifts be the same? Or is there some reason the day shift should have more failures?
This analysis does not consider whether or not the failure rates were constant throughout each shift.
If the failure rate is changing throughout a shift, what do the counts above represent?
If the difference between the shifts persists over time, then there might be a systematic reason for the difference. The analysis above merely assumes that the failure rates were constant and proceeds to draw a conclusion that the shifts WERE different. This is no guarantee that they will be different tomorrow.
Both the nature of the inference and the quality of the information provided by this analysis is fundamentally different from that provided by process behavior charts.
On those rare occasions when you can safely assume the data are homogeneous, the traditional approaches make sense. In all other cases let the user beware.
While the chi-square test can be extended to categorical data for three or more conditions, the same issues apply. If we have temporal order, put the data on XmR charts by category and condition. If we do not have temporal order, we have nothing more than an enumerative study which may or may not predict anything.
"
"
It is all in making the distinction between
What is?
and
Why it is?
and
Whether it will stay that way?
"
The strong argument is demonstrated very simply.
Good afternoon, Tony
Excellent article. The strong argument is demonstrated very simply.
I fully support your attitude toward analytical research with the help of the Shewhart control charts
Yours faithfully,
Sergey Grigoryev, DEMING.PRO
Thank you Sergey.
Thank you Sergey.
enumerative and analytic methods
Dear Dr. Burns,
This is one of the powerful articles that enlighten anyone about the importance of using tools. But as just begginner in stats, could you please shed light about what are enumerative tools and analytics tools? Is the SPC only considered as analtics tools? Where to start to my study about the topics?
Thanks in advance for your help
The difference between analytic and enumerative studies
In the earliest reference for which I am aware, Deming proposed the differences between the two types of studies in his seminal work on curve-fitting, Some Theory of Sampling. You can still find Dover editions of that book.
Deming stated that the difference between the two types of studies is the intent. Essentially, enumerative studies deal with a population. You are sampling (collecting statistics) from the population to estimate its parameters, with the intention of taking action on some aspect of the population. The data are static: At least in principle you know every member of the population and can attribute any characteristics of those members to the moment in time when you drew the sample. It is like examining a snapshot with a lot of holes in it, using what you can glean from the information you have to fill in those holes and estimate what the whole picture would look like.
Analytic studies are intended for action on a cause system. They are not static, but dynamic. In an analytic study there is no population of interest. We are trying to sample from the past or present, and studying those data to try to extrapolate into the future. It's a little like watching a movie and looking at what's causing the current actions on the screen, and using that information to try to figure out what will happen next.
Here's where it gets tricky (example courtesy of David Kerridge): In Out of the Crisis, Deming used an example to illustrate operational definitions. It was a destructive testing example to test whether a blanket was 50% wool. In the operational definition, he outlines a test procedure where the analyst punches 10 holes one inch in diameter, centered by random numbers, then tests the content of that sample. If the sample proves to be greater than 50% wool (plus or minus 2% if I remember right), then the blanket can be considered to be "50% wool."
So, that test would be an enumerative study...the "population" is all the fabric in the blanket, the sample comprises the circles punched from the wool, and we are extrapolating from those circles to the remainder of the blanket. It would be appropriate, in this case, to compute at least a confidence interval around the average wool content from the sample.
However suppose this test were conducted at some regular time interval...say, once or twice per day. If we use the numbers to run an averages and ranges chart (or an individuals and ranges chart) to get the average and control limits over time (to look for special causes or trends), we have used our enumerative study results to get data for an analytic study. If we were doing that, then we would just get the average wool content from each sample, and not run any sort of confidence interval or t-test.
Hopefully this helps, Mohamed. If you've read the rest of this discussion, you can see that there is (lamentably) little in the literature any more about this topic.
Add new comment