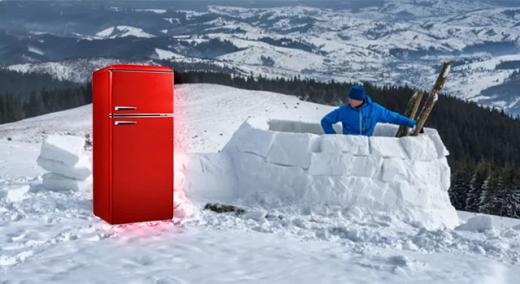
There is a type of error that occurs when conducting statistical testing: to work very hard to correctly answer the wrong question. This error occurs during the formation of the experiment.
ADVERTISEMENT |
Despite creating a perfect null and alternative hypothesis, sometimes we are simply investigating the wrong question.
Example of a type III error
Let’s say we really want to select the best vendor for a critical component of our design. We define the best vendor as one whose solution or component is the most durable. OK, we can set up an experiment to determine which vendor provides a solution that is the most durable.
We set up and conduct a flawless hypothesis test to compare the two leading solutions. We can see very clear results. Vendor A’s solution is, statistically, significantly more durable than Vendor B’s solution.
Yet neither solution is durable enough. We should have been evaluating if either solution could meet our reliability requirements instead.
Oops.
Even if we perfectly answer a question in our work, if it’s not the right question, then the work is for naught.
…
Comments
The Right Question
The quote from Einstein comes to mind: "If I had an hour to solve a problem and my life depended on it, I would use the first 55 minutes determining the proper question to ask, for once I know the proper question, I could solve the problem in less than five minutes. Einstein
Asking questions is difficult, especially inquiry based questions that produce useful predictions (theory). A great challenge in the use of PDSA. When I saw the word, "Significance," Deming's Forward to Quality Improvement through Planned Experimentation came to mind:
This book by Ronald D. Moen, Thomas W. Nolan, and Lloyd Provost breaks new ground in the problem of prediction based on data from comparisons of two or more methods or treatments, tests of materials, and experiments.
Why does anyone make a comparison of two methods, two treatments, two processes, or two materials? Why does anyone carry out a test or an experiment? The answer is to predict—to predict whether one of the methods or materials tested will in the future, under a specified range of conditions, performs better than the other one.
Prediction is the problem, whether we are talking about applied science, research and development, engineering, or management in industry, education, or government. The question is, What do the data tell us? How do they help us to predict?
Unfortunately, the statistical methods in textbooks and in the classroom do not tell the student that the problem in the use of data is prediction. What the student learns is how to calculate a variety of tests (t-test, F-test, chi-square, goodness of fit, etc.) in order to announce that the difference between the two methods or treatments is either significant or not significant. Unfortunately, such calculations are a mere formality. Significance or the lack of it provides no degree of belief—high, moderate, or low—about prediction of performance in the future, which is the only reason to carry out the comparison, test, or experiment in the first place.
Any symmetric function of a set of numbers almost always throws away a large portion of the information in the data. Thus, interchange of any two numbers in the calculation of the mean of a set of numbers, their variance, or their fourth moment does not change the mean, variance, or fourth moment. A statistical test is a symmetric function of the data.
In contrast, interchange of two points in a plot of points may make a big difference in the message that the data are trying to convey for prediction.
The plot of pints conserves the information derived from the comparison or experiment. It is for this reason that the methods taught in this book are a major contribution to statistical methods as an aid to engineers, as well as to those in industry, education, or government who are trying to understand the meaning of figures derived from comparisons or experiments. The authors are to be commended for their contributions to statistical methods.
W. Edwards Deming
Washington, July 14, 1990
Type III and Higher Errors
My personal experience is that I find many Type III errors (my version of Type III) originate from observational studies versus designed experiments. Modern data analytics often executes computationally massive observational statistical significance fishing expeditions but does not account for the total number of implicit hypotheses being tested in making the significance judgement, the implications of data structure nor the implications of failing to approximately represent an appropriate distributional error model. Designed experiments choose the focus of the study and somewhat structure the data collection, cutting down on excessively obtaining higher numbers of spurious results or correlations. Enjoyable discussion, thanks for publishing it.
Add new comment