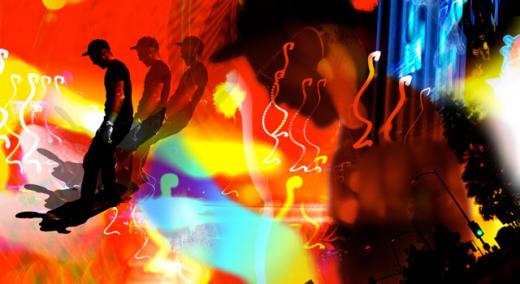
"Temperature" Credit:Feel Mystic
The keys to effective process behavior charts are rational sampling and rational subgrouping. As implied by the word rational, we must use our knowledge of the context to collect and organize data in a way that answers the interesting questions. This column will show the role that sample frequency plays in constructing an effective XmR chart.
ADVERTISEMENT |
One of my clients had an online temperature gauge that could be sampled at different frequencies. The process engineer wanted to use these data to create a process behavior chart. He began by sampling the temperature once every 128 seconds, resulting in a sample frequency of 28 times per hour. The resulting XmR chart is shown in figure 1.
Figure 1: Temperature sampled 28 times per hour
…
Comments
Rational Sampling
The Process Engineer's project mentioned in this article reminded me of a 6-Sigma Black Belt project that I helped advise in a paper mill about 10 years ago. I came into the project after the data collection phase and was reviewing the Process Behavior Charts with the Process Engineer after he had collected about two weeks' worth of data. Initially, the charts appeared to be full of special causes; the charts had no semblance of stability. However, after asking a few questions, it turned out that the data points were only 5 seconds apart in their measurement! The data consisted of temperature readings of a pulp slurry exiting a 10,000 gallon tank at a rate of about 50 gallons per minute. The tank had a powerful agitator in it to keep the consistency of the slurry as even as possible. There was, indeed, a consistency meter in the pipeline exiting the tank. We charted the consistency data and found the consistency measurements to be wild as well. The problem was quickly recognized as "rational sampling". There was none! Upon reflection, it became obvious that a 10,000 gallon tank with a thoughput of 50 gpm, cannot possibly experience "real" or "practical" temperature or consistency changes in 5 seconds. An analysis to the autocorrelation of these two variables confirmed that the autocorrelation coefficient was greater than 85%. Thus, the sampling (every 5 seconds) was confirmed to be not rational. The problem was corrected by taking an average of 5 readings taken within a 1 minute span of time (randomly selected from the every 5 seconds data). The averages were taken every 15 minutes. Data collected in this manner showed a stable, predictable process with reasonable control limits, allowing for an 80% reduction in operator adjustments to the process.
As always, you explain complex things in simple language.
Thanks for the article dear Dr. Donald J. Wheeler.
As always, you explain complex things in simple language.
Thank you for sharing your knowledge with the world!
May God grant you long life!
Rational sampling
Always a pleasure to read !
Thank you so much!
Sampling too infrequently for high speed, unpredictable processe
Dr. Wheeler,
Thank you again for helping many of us setup and use process behavior charts effectively. While in this article you highlighted an example of the effects of too frequent sampling, you do mention the ills of too infrequent sampling of unprecitable processes -- specifically that such infrequent sampling can inadvertently cause the process behavior to look more predictable.
While you say "generally, in between frequencies that are too low and frequencies that are too high, there will be some region where different sampling frequencies will result in similar limits", do you really often see that when frequencies are too low to start with for an unpredictable process?
It seems logical and it has been my experience that unpredictable processes that run really fast but are sampled really infrequently (due to cost of labor) the limits will get narrower and narrower as the sampling frequency is increased over and over. And they are likely giving true, not false evidence of trouble in the process. Perhaps I just never got down to anything close to sampling every successive part, the fastest sampling possible.
It seems the concept of a process time constant would be worthy here. That and the degree of unpredictability in the process are factors in that judgement you mention? Faster more unpredictable processes can benefit from sampling more frequently and so on. But then, we have the problem you've written about elsewhere. If the current sampling frequency is already giving signals and if we aren't taking advantage of that information, why in the world would we need more signals from a faster frequency?
response for Blaine Kelly
Thanks for raising this question.
Rational sampling combines the context for the process and the purpose ofr the charts. It always requires judgment about what kind of process changes might occur and how fast they might show up in the data. Remember, we are not concerned with every little process change, but only those that are large enough to be of economic consequence.
I recommend starting with a higher sample frequency rather than a lower for exactly the reasons you note. However, when we reach a frequency that will allow the subgroup ranges, or the moving ranges, to capture the routine process variation the limits will stabilize. If the limits simply continue to shrink with increasing frequencies your initial frequency may have been too high.
When the limits stabilize you have empirical evidence that you have the right frequency. This empirical evidence should be used along with the context to get useful charts. When confronted with a process that produces data at a high frequency I tend to start with the running recod and look at the overall bandwidth of that record. Is it steady or does it meander around? This is just one more empirical trick to help with the judgment involved in rational sampling. As my colleague Richard Lyday used to say, we should always think first, then think statistically.
So keep asking questions.
Add new comment